Documents
Presentation Slides
LOFT: LATENT SPACE OPTIMIZATION AND GENERATOR FINE-TUNING FOR DEFENDING AGAINST DEEPFAKES
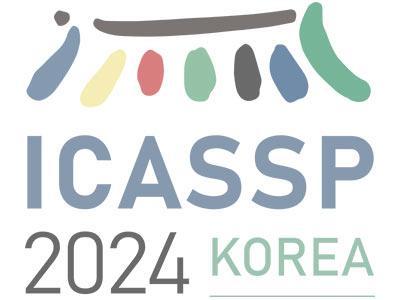
- DOI:
- 10.60864/4eev-h149
- Citation Author(s):
- Submitted by:
- Shaoyou Zeng
- Last updated:
- 13 April 2024 - 2:54am
- Document Type:
- Presentation Slides
- Categories:
- Log in to post comments
DeepFakes pose a significant threat to individual reputations and society as a whole. Existing proactive defense strategies concentrate on adding adversarial perturbations to images to disrupt or nullify the generation of DeepFakes, but these approaches are easily detectable by human perception and can be removed. To address this challenge, we propose a three-stage framework called LOFT (Latent Space Optimization and Generator Fine-Tuning for Defending against DeepFakes). First, encoding the original image into the latent space to obtain a latent code that captures facial features. Second, utilizing Adversarial Latent Optimization to optimize the latent code for reconstructing the image and defending against DeepFake manipulation. Third, fine-tuning the generator to enhance the reconstructed image's visual quality and defense capability further. Our study evaluates the effectiveness of our proposed framework through two distinct DeepFake tasks: attribute editing and face reenactment. Various experimental results demonstrate that our proposed framework outperforms the existing benchmark in both visual quality and defense capability.