Documents
Presentation Slides
Look globally, age locally: Face aging with an attention mechanism
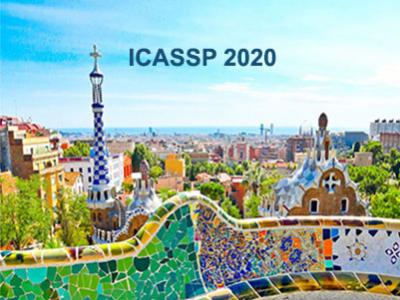
- Citation Author(s):
- Submitted by:
- Haiping Zhu
- Last updated:
- 15 May 2020 - 9:17pm
- Document Type:
- Presentation Slides
- Document Year:
- 2020
- Event:
- Presenters:
- Haiping Zhu
- Paper Code:
- IVMSP-L6.4
- Categories:
- Log in to post comments
Face aging is of great importance for cross-age recognition and entertainment-related applications. Recently, conditional generative adversarial networks (cGANs) have achieved impressive results for facial aging. Existing cGANs-based methods usually require a pixel-wise loss to keep the identity and background consistent. However, minimizing the pixel-wise loss between the input and synthesized images likely resulting in a ghosted or blurry face. To address this deficiency, this paper introduces an Attention Conditional GANs (AcGANs) approach for facial aging, which utilizes attention mechanism to \emph{only} alert the regions relevant to face aging. In doing so, the synthesized face can well preserve the background information and personal identity without using the pixel-wise loss, and the ghost artifacts and blurriness can be significantly reduced. Based on the benchmarked dataset Morph, both qualitative and quantitative experiment results demonstrate superior performance over existing algorithms in terms of image quality, personal identity, and age accuracy. Codes are available on \href{https://github.com/JensonZhu14/AcGAN}{https://github.com/JensonZhu14/AcGAN}.
Comments
A presentation slice for the
A presentation slice for the paper ``Look globally, age locally: Face aging with an attention mechanism'', which has been published in ICASSP2020.