Documents
Presentation Slides
LOW-RANK AND SPARSE DECOMPOSITION FOR JOINT DOA ESTIMATION AND CONTAMINATED SENSORS DETECTION WITH SPARSELY CONTAMINATED ARRAYS
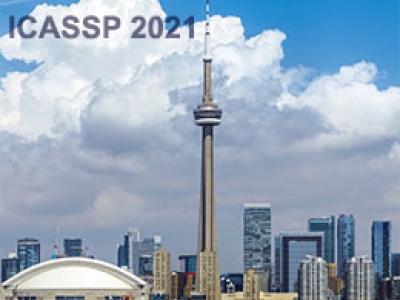
- Citation Author(s):
- Submitted by:
- huiping huang
- Last updated:
- 22 June 2021 - 2:56am
- Document Type:
- Presentation Slides
- Document Year:
- 2021
- Event:
- Presenters:
- Huiping Huang
- Paper Code:
- SAM-10.5
- Categories:
- Log in to post comments
Many works have been done in direction-of-arrival (DOA) estimation in the presence of sensor gain and phase uncertainties in the past decades. Most of the existing approaches require either auxiliary sources with exactly known DOAs or perfectly partly calibrated arrays. In this work, we consider sparsely contaminated arrays in which only a few sensors are contaminated by sensor gain and phase errors, and moreover, the number of contaminated sensors as well as their positions are unknown. Such arrays exist in many real-world scenarios, and it can be regarded as a general case of the partly calibrated arrays, in which the number and positions of calibrated (or uncontaminated) sensors are known a priori. Based on the sparsity of sensor errors, we formulate the DOA estimation problem under the framework of low-rank and sparse decomposition. We develop an iteratively reweighted least squares method to solve the resulting problem. Our methods can estimate the DOAs of incoming signals and detect the contaminated sensors simultaneously. Numerical results exhibit the effectiveness and superiority of the proposed methods in both DOA estimation and contaminated sensors detection.