Documents
Poster
Machine Load Estimation via Stacked Autoencoder Regression
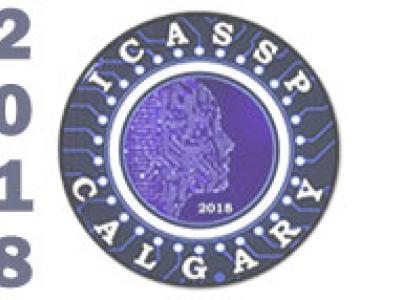
- Citation Author(s):
- Submitted by:
- TULIKA BOSE
- Last updated:
- 13 April 2018 - 4:20am
- Document Type:
- Poster
- Document Year:
- 2018
- Event:
- Presenters:
- Tulika Bose
- Paper Code:
- IDSP-P1.2
- Categories:
- Keywords:
- Log in to post comments
The problem of load estimation from sensor signals holds significance in the field of intelligent manufacturing. The goal of this work is to estimate the axial and spindle load values in a Computer Numerical Control machine from input sensor readings like spindle speed, feed rate, tool positions, etc. This can be viewed as a standard regression problem. Here, we propose a novel deep learning based regression technique that incorporates regression within the stacked autoencoder framework. Unlike the popular heuristic pretraining, fine-tuning approach, we solve all the parameters of the problem jointly. A variable splitting Augmented Lagrangian approach is employed to solve the ensuing optimization problem. Comparisons on standard regression models like linear, Least Absolute Shrinkage and Selection Operator (LASSO), Support Vector Regression and the traditional stacked autoencoder have shown that our technique considerably outperforms them.