Documents
Poster
MDX-GAN: ENHANCING PERCEPTUAL QUALITY IN MULTI-CLASS SOURCE SEPARATION VIA ADVERSARIAL TRAINING
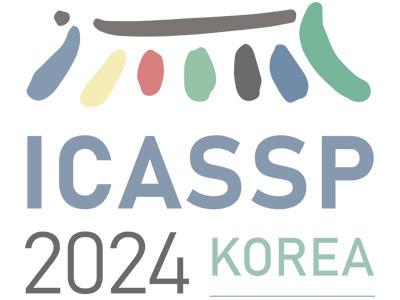
- Citation Author(s):
- Submitted by:
- Ke Chen
- Last updated:
- 15 April 2024 - 8:27am
- Document Type:
- Poster
- Document Year:
- 2024
- Event:
- Presenters:
- Ke Chen
- Paper Code:
- AASP-P16.6
- Categories:
- Log in to post comments
Audio source separation aims to extract individual sound sources from an audio mixture. Recent studies on source separation focus primarily on minimizing signal-level distance, typically measured by source-to-distortion ratio (SDR). However, scant attention has been given to the perceptual quality of the separated tracks. In this paper, we propose MDX-GAN, an efficient and high-fidelity audio source separator based on MDX-Net for multiple sound classes. We leverage different training objectives to enhance the perceptual quality of audio source separation. Specifically, we adopt perceptually-motivated loss functions on top of the waveform loss, including multi-resolution STFT and Mel-spectrogram losses, and employ the adversarial training paradigm with multi-domain and multi-scale discriminators to refine the perceptual quality of separation. Additionally, we extend the model to support multiple sound classes within a single network via feature-wise linear modulation (FiLM). We conduct both objective and subjective experiments to evaluate MDX-GAN on real-world settings, and assess the impacts of design components on the perceptual quality and SDR scores. Results demonstrate that MDX-GAN accurately separates the sound source and achieves superior perceptual quality.