Documents
Poster
MEMORY-AUGMENTED DUAL-DOMAIN UNFOLDING NETWORK FOR MRI RECONSTRUCTION
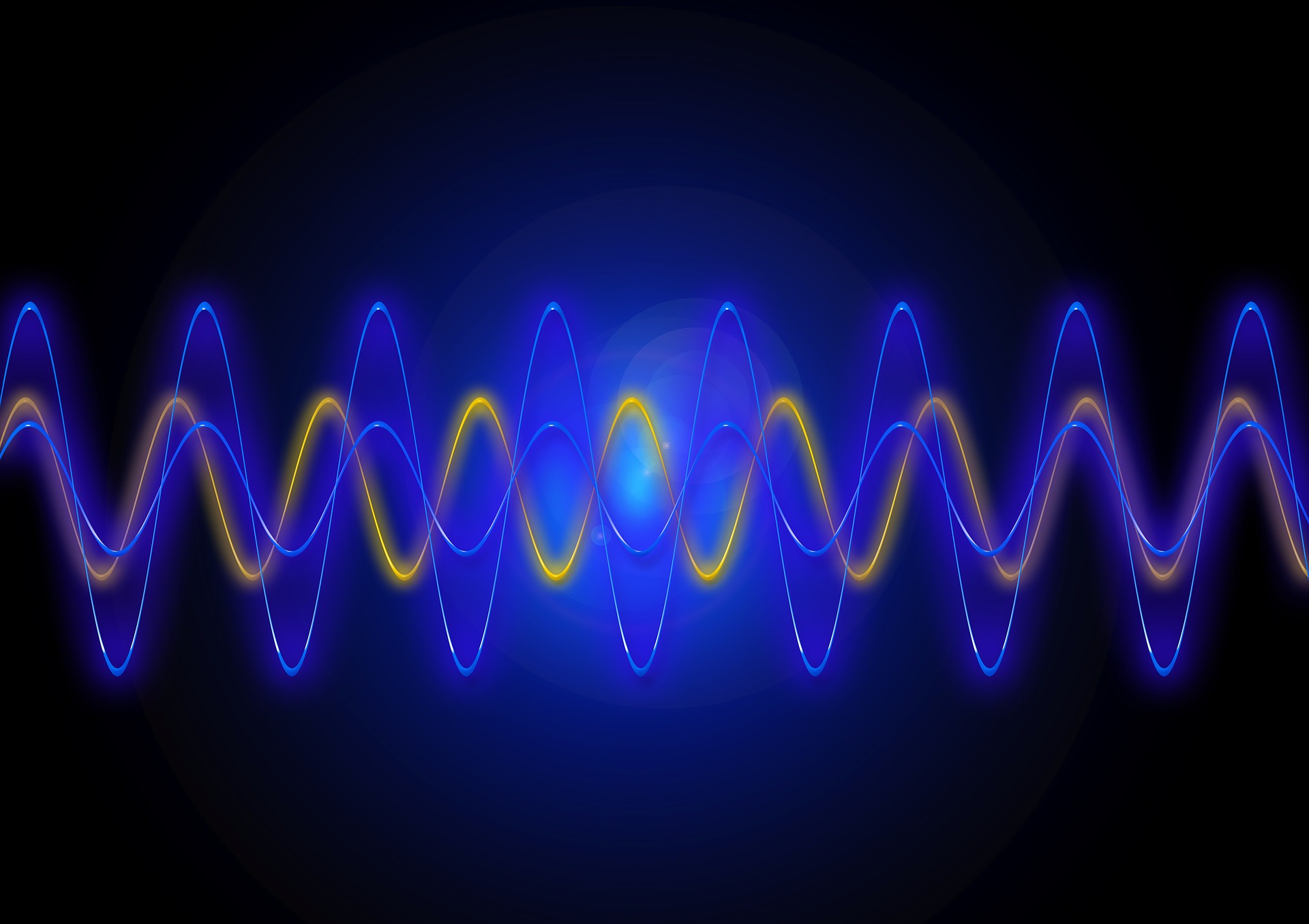
- DOI:
- 10.60864/0xwy-jc90
- Citation Author(s):
- Submitted by:
- Jiawei Jiang
- Last updated:
- 6 June 2024 - 10:54am
- Document Type:
- Poster
- Categories:
- Log in to post comments
The compressed sensing MRI aims to recover high-fidelity images from undersampled k-space data, which enables MRI acceleration and meanwhile mitigates problems caused by prolonged acquisition time, such as physiological motion artifacts, patient discomfort, and delayed medical care. In this regard, the deep unfolding network (DUN) has emerged as the predominant solution due to the benefits of better interpretability and model capacity. However, existing algorithms remain inadequate for two principal reasons. First, directly unrolling a typical optimization algorithm is ill-considered for the structure information and domain knowledge.
Second, the incorporation of the MRI-oriented imaging mechanism is inadequate. To tackle these two issues, we propose a Memoryaugmented Dual-domain Unfolding Network (MDUNet). Particularly, the per-iteratively learned memory is held to facilitate a better efficacy of feature representation. Besides, with the scheme of memory augmentation alternatively employed in the k-space and image
domain, both the regional structure and global information can be complementarily integrated in a spiral manner. Comprehensive experiments conducted on diverse datasets, sampling rates, and sampling patterns demonstrate that our method, while maintaining a relatively small number of parameters, surpasses the latest methods.