Documents
Poster
MODALITY-DEPENDENT SENTIMENTS EXPLORING FOR MULTI-MODAL SENTIMENT CLASSIFICATION
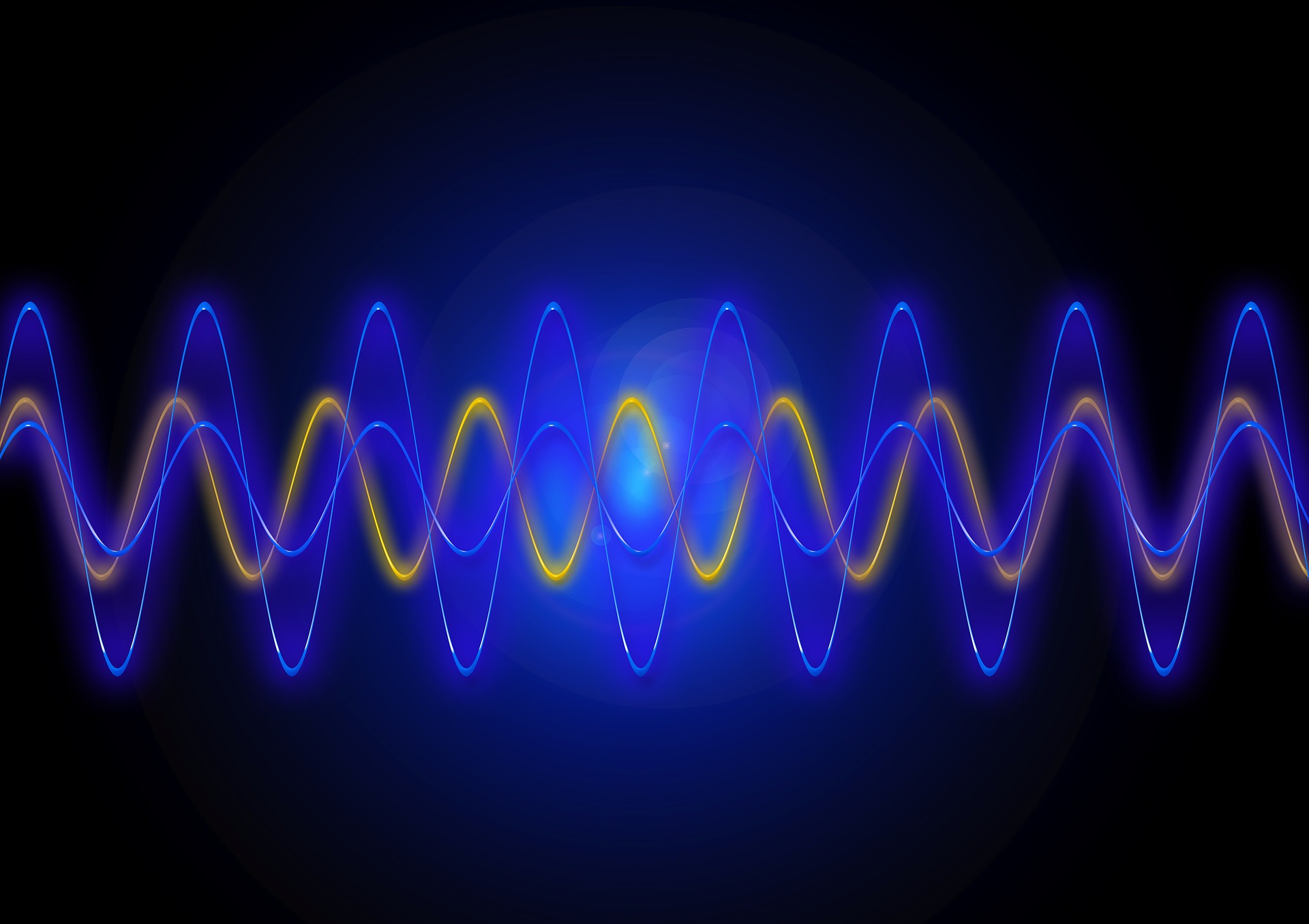
- DOI:
- 10.60864/znx5-vs32
- Citation Author(s):
- Submitted by:
- Jingzhe Li
- Last updated:
- 6 June 2024 - 10:50am
- Document Type:
- Poster
- Categories:
- Log in to post comments
Recognizing human feelings from image and text is a core
challenge of multi-modal data analysis, often applied in personalized advertising. Previous works aim at exploring the
shared features, which are the matched contents between
images and texts. However, the modality-dependent sentiment information (private features) in each modality is usually ignored by cross-modal interactions, the real sentiment
is often reflected in one modality. In this paper, we propose a Modality-Dependent Sentiment Exploring framework
(MDSE). First, to exploit the private features, we compare
shared features with original image or text features, identifying previously overlooked unimodal features. Fusing the
private and shared features can make the model more robust.
Second, in order to obtain unified sentiment representations,
we treat unimodal features and multi-modal fused features
equally. We introduce a Modality-Agnostic Contrastive Loss
(MACL) that performs contrastive learning between unimodal features and multi-modal fused features. The MACL
can fully exploit sentiment information from multi-modal
data and reduce the modality gap. Experiments on four
public datasets demonstrate the effectiveness of our MDSE
compared with existing methods. The full codes are available
at https://github.com/royal-dargon/MDSE.