Documents
Presentation Slides
Multi-Conditioning & Data Augmentation using Generative Noise Model for Speech Emotion Recognition in Noisy Conditions
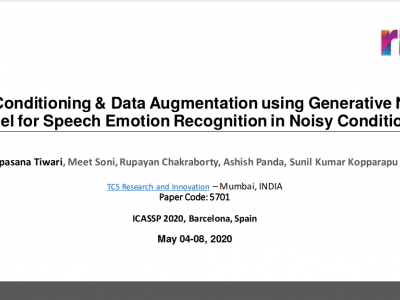
- Citation Author(s):
- Submitted by:
- Upasana Tiwari
- Last updated:
- 20 May 2020 - 5:01am
- Document Type:
- Presentation Slides
- Document Year:
- 2020
- Event:
- Presenters:
- Upasana Tiwari
- Paper Code:
- 5701
- Categories:
- Keywords:
- Log in to post comments
Degradation due to additive noise is a significant road block in the real-life deployment of Speech Emotion Recognition (SER) systems. Most of the previous work in this field dealt with the noise degradation either at the signal or at the feature level. In this paper, to address the robustness aspect of the SER in additive noise scenarios, we propose multi-conditioning and data augmentation using an utterance level parametric generative noise model. The generative noise model is designed to generate noise types which can span the entire noise space in the mel-filterbank energy domain. This characteristic of the model renders the system robust against unseen noise conditions. The generated noise types can be used to create multi-conditioned data for training the SER systems. Multi-conditioning approach can also be used to increase the training data by many folds where such data is limited. We report the performance of the proposed method on two datasets, namely EmoDB and IEMOCAP. We also explore multi-conditioning and data augmentation using noise samples from NOISEX-92 noise database.