- Acoustic Modeling for Automatic Speech Recognition (SPE-RECO)
- General Topics in Speech Recognition (SPE-GASR)
- Large Vocabulary Continuous Recognition/Search (SPE-LVCR)
- Lexical Modeling and Access (SPE-LEXI)
- Multilingual Recognition and Identification (SPE-MULT)
- Resource constrained speech recognition (SPE-RCSR)
- Robust Speech Recognition (SPE-ROBU)
- Speaker Recognition and Characterization (SPE-SPKR)
- Speech Adaptation/Normalization (SPE-ADAP)
- Speech Analysis (SPE-ANLS)
- Speech Coding (SPE-CODI)
- Speech Enhancement (SPE-ENHA)
- Speech Perception and Psychoacoustics (SPE-SPER)
- Speech Production (SPE-SPRD)
- Speech Synthesis and Generation, including TTS (SPE-SYNT)
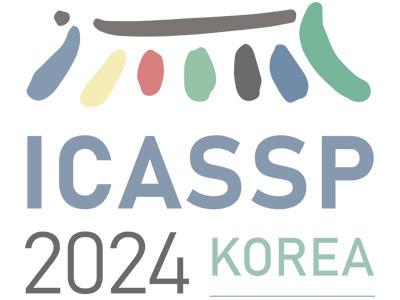
- Read more about LEVERAGING EFFECTIVE LANGUAGE AND SPEAKER CONDITIONING IN INDIC TTS FOR LIMMITS 2024 CHALLENGE
- Log in to post comments
In this paper, we explain the model that was developed by the NLP\_POSTECH team for the LIMMITS 2024 Grand Challenge. Among the three tracks, we focus on Track 1, which necessitates the creation of a few-shot text-to-speech (TTS) system that generates natural speech across diverse languages. Towards this end, to realize multi-lingual capability, we incorporate a learnable language embedding. In addition, for precise imitation of target speaker voices, we leverage an inductive speaker bias conditioning methodology.
- Categories:

- Read more about Presentation of Diffusion-based speech enhancement with a weighted generative-supervised learning loss
- 1 comment
- Log in to post comments
Diffusion-based generative models have recently gained attention in speech enhancement (SE), providing an alternative to conventional supervised methods. These models transform clean speech training samples into Gaussian noise, usually centered on noisy speech, and subsequently learn a parameterized
- Categories:
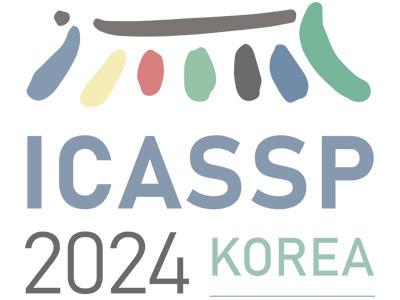
- Read more about LEVERAGING EFFECTIVE LANGUAGE AND SPEAKER CONDITIONING IN INDIC TTS FOR LIMMITS 2024 CHALLENGE
- Log in to post comments
In this paper, we explain the model that was developed by the NLP\_POSTECH team for the LIMMITS 2024 Grand Challenge. Among the three tracks, we focus on Track 1, which necessitates the creation of a few-shot text-to-speech (TTS) system that generates natural speech across diverse languages. Towards this end, to realize multi-lingual capability, we incorporate a learnable language embedding. In addition, for precise imitation of target speaker voices, we leverage an inductive speaker bias conditioning methodology.
- Categories:
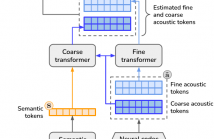
- Read more about Speaker anonymization using neural audio codec language models
- Log in to post comments
The vast majority of approaches to speaker anonymization involve the extraction of fundamental frequency estimates, linguistic features and a speaker embedding which is perturbed to obfuscate the speaker identity before an anonymized speech waveform is resynthesized using a vocoder.
Recent work has shown that x-vector transformations are difficult to control consistently: other sources of speaker information contained within fundamental frequency and linguistic features are re-entangled upon vocoding, meaning that anonymized speech signals still contain speaker information.
- Categories:
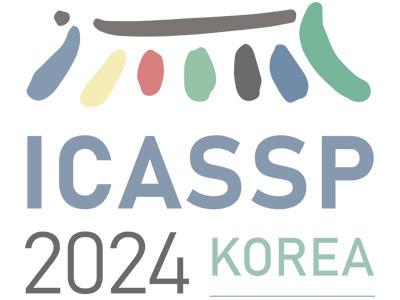
- Read more about CONTEXTUAL BIASING OF NAMED-ENTITIES WITH LARGE LANGUAGE MODELS
- Log in to post comments
We explore contextual biasing with Large Language Models (LLMs) to enhance Automatic Speech Recognition (ASR) in second-pass rescoring. Our approach introduces the utilization of prompts for LLMs during rescoring without the need for fine-tuning. These prompts incorporate a biasing list and a set of few-shot examples, serving as supplementary sources of information when evaluating the hypothesis score. Furthermore, we introduce multi-task training for LLMs to predict entity class and the subsequent token.
- Categories:
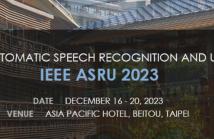
- Read more about PSEUDO-LABEL BASED SUPERVISED CONTRASTIVE LOSS FOR ROBUST SPEECH REPRESENTATIONS
- 1 comment
- Log in to post comments
The self supervised learning (SSL) of speech, with discrete tokenization (pseudo-labels), while illustrating performance improvements in low-resource speech recognition, has faced challenges in achieving context invariant and noise robust representations. In this paper,we propose a self-supervised framework based on contrastive loss of the pseudo-labels, obtained from an offline k-means quantizer (tokenizer). We refer to the proposed setting as pseudo-con.
- Categories:
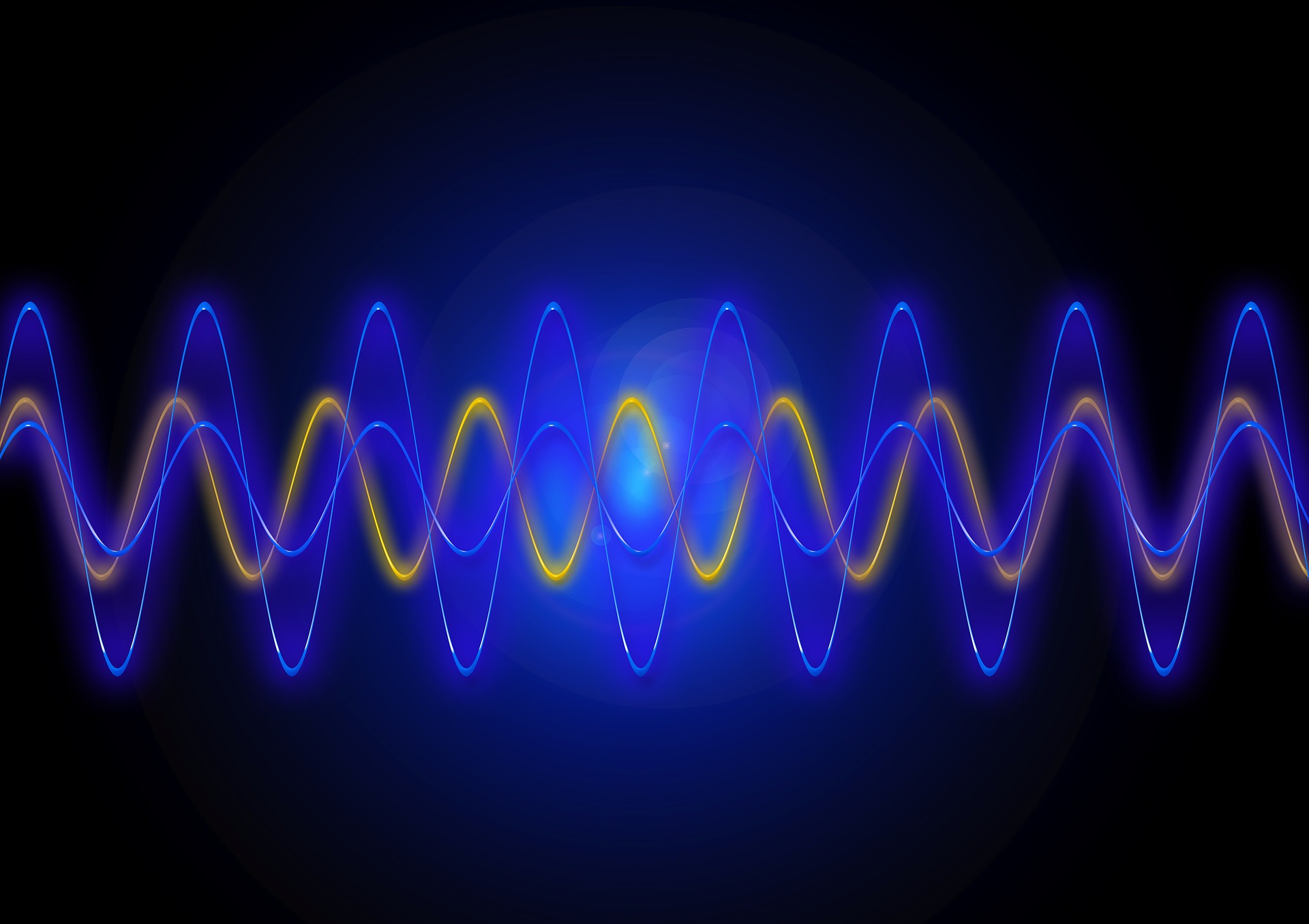
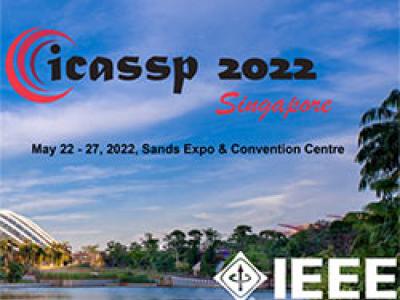
- Read more about On Language Model Integration for RNN Transducer based Speech Recognition
- Log in to post comments
The mismatch between an external language model (LM) and the implicitly learned internal LM (ILM) of RNN-Transducer (RNN-T) can limit the performance of LM integration such as simple shallow fusion. A Bayesian interpretation suggests to remove this sequence prior as ILM correction. In this work, we study various ILM correction-based LM integration methods formulated in a common RNN-T framework. We provide a decoding interpretation on two major reasons for performance improvement with ILM correction, which is further experimentally verified with detailed analysis.
- Categories:
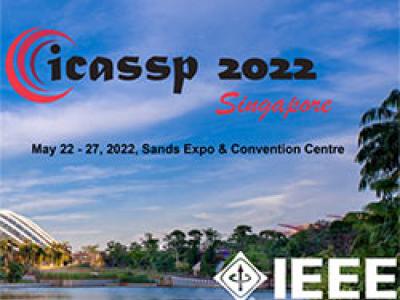
- Read more about VarianceFlow: High-quality and Controllable Text-to-Speech Using Variance Information via Normalizing Flow
- Log in to post comments
- Categories:
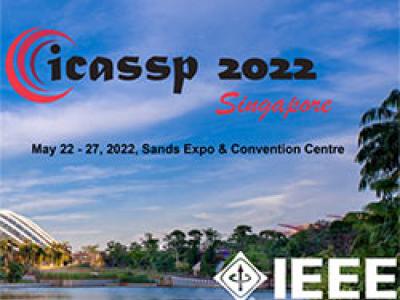
- Read more about VarianceFlow: High-quality and Controllable Text-to-Speech Using Variance Information via Normalizing Flow
- Log in to post comments
- Categories: