Documents
Presentation Slides
Multi-Density Convolutional Neural Network for In-Loop Filter in Video Coding
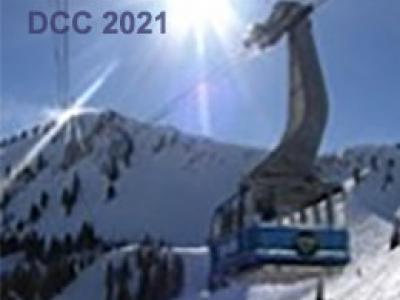
- Citation Author(s):
- Submitted by:
- Zhao Wang
- Last updated:
- 28 February 2021 - 3:57am
- Document Type:
- Presentation Slides
- Event:
- Presenters:
- Zhao Wang
- Paper Code:
- 114
- Categories:
- Log in to post comments
As the latest video coding standard, Versatile Video Coding (VVC) achieves up to 40% Bjøntegaard delta bit-rate (BD-rate) reduction compared with High Efficiency Video Coding (HEVC). Recently, Convolutional Neural Network (CNN) has attracted tremendous attention and shows great potential in video coding. In this paper, we design a Multi-Density Convolutional Neural Network (MDCNN) as an integrated in-loop filter to improve the quality of the reconstructed frames. The core of our approach is the multi-density block (MDB), which contains two branches: (a) the basic branch maintaining full resolution for capturing spatially-precise representations, (b) the density branch for learning rich spatial correlation with larger receptive field through down-sampling and up-sampling. The feature maps of two branches will be fused into one stream repeatedly. Benefiting from this architecture, spatially-precise representations and density correlations in larger receptive field are utilized to improve the model performance and promote the model’s robustness to different input resolutions. Experimental results show that, in terms of BD-rate savings for the (Y, U, V) components compared to the state-of-the-art VVC standard, the proposed MDCNN filter can achieve (5.06%, 13.86%, 13.76%) and (4.36%, 10.85%, 10.91%) coding gain for the Random Access (RA) configuration and the All Intra (AI) configuration, respectively.
DCC-114.pptx
