Documents
Poster
Multi-frame Full-rank Spatial Covariance Analysis for Underdetermined BSS in Reverberant Environment
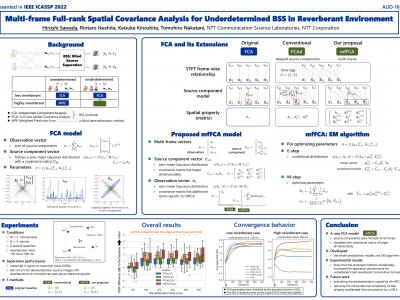
- Citation Author(s):
- Submitted by:
- Hiroshi Sawada
- Last updated:
- 11 May 2022 - 3:28am
- Document Type:
- Poster
- Document Year:
- 2022
- Event:
- Presenters:
- Hiroshi Sawada
- Paper Code:
- AUD16-1
- Categories:
- Log in to post comments
Full-rank spatial covariance analysis (FCA) is a blind source separation (BSS) method, and can be applied to underdetermined cases where the sources outnumber the microphones. This paper proposes a new extension of FCA, aiming to improve BSS performance for mixtures in which the length of reverberation exceeds the analysis frame. There has already been proposed a model that considers delayed source components as the exceeded parts. In contrast, our new extension models multiple time frames with multivariate Gaussian distributions of larger dimensionality than the existing FCA models. We derive an expectation-maximization algorithm to optimize the model parameters. Experiments to separate four speech sources with three microphones show that the proposed extension outperforms the existing models, and more specifically, the original FCA by around 2 dB measured with signal-to-distortion ratio.