Documents
Poster
MULTI-LEVEL CONTRASTIVE LEARNING FOR CROSS-LINGUAL ALIGNMENT
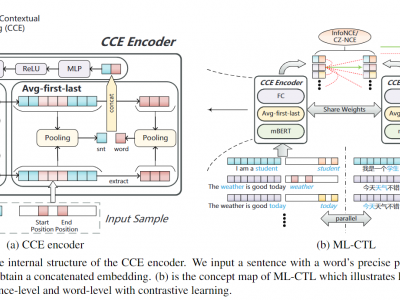
- Citation Author(s):
- Submitted by:
- Beiduo Chen
- Last updated:
- 5 May 2022 - 10:34am
- Document Type:
- Poster
- Document Year:
- 2022
- Event:
- Presenters:
- Beiduo Chen
- Paper Code:
- SPE-67.5
- Categories:
- Log in to post comments
Cross-language pre-trained models such as multilingual BERT (mBERT) have achieved significant performance in various cross-lingual downstream NLP tasks. This paper proposes a multi-level contrastive learning (ML-CTL) framework to further improve the cross-lingual ability of pre-trained models. The proposed method uses translated parallel data to encourage the model to generate similar semantic embeddings for different languages. However, unlike the sentence-level alignment used in most previous studies, in this paper, we explicitly integrate the word-level information of each pair of parallel sentences into contrastive learning. Moreover, cross-zero noise contrastive estimation (CZ-NCE) loss is proposed to alleviate the impact of the floating-point error in the training process with a small batch size. The proposed method significantly improves the cross-lingual transfer ability of our basic model (mBERT) and outperforms on multiple zero-shot cross-lingual downstream tasks compared to the same-size models in the Xtreme benchmark.