Documents
Presentation Slides
Multi-step Online Unsupervised Domain Adaptation
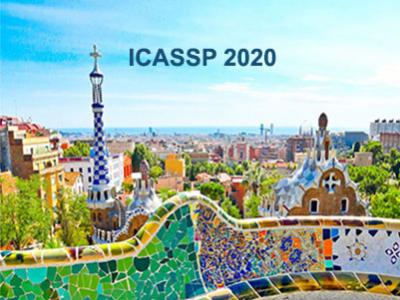
- Citation Author(s):
- Submitted by:
- Jihoon Moon
- Last updated:
- 14 May 2020 - 11:58pm
- Document Type:
- Presentation Slides
- Event:
- Presenters:
- Jihoon Moon
- Paper Code:
- MLSP-P14.3
- Categories:
- Log in to post comments
In this paper, we address the Online Unsupervised Domain Adaptation (OUDA) problem, where the target data are unlabelled and arriving sequentially. The traditional methods on the OUDA problem mainly focus on transforming each arriving target data to the source domain, and they do not sufficiently consider the temporal coherency and accumulative statistics among the arriving target data. We propose a multi-step framework for the OUDA problem, which institutes a novel method to compute the mean-target subspace inspired by the geometrical interpretation on the Euclidean space. This mean-target subspace contains accumulative temporal information among the arrived target data. Moreover, the transformation matrix computed from the mean-target subspace is applied to the next target data as a preprocessing step, aligning the target data closer to the source domain. Experiments on four datasets demonstrated the contribution of each step in our proposed multi-step OUDA framework and its performance over previous approaches.