Documents
Poster
MULTIMODAL IMAGING FEATURE EXTRACTION WITH REFERENCE CANONICAL CORRELATION ANALYSIS UNDERLYING INTELLIGENCE
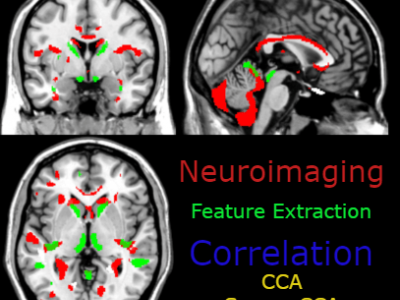
- DOI:
- 10.60864/4t8z-s327
- Citation Author(s):
- Submitted by:
- RAM SAPKOTA
- Last updated:
- 6 June 2024 - 10:33am
- Document Type:
- Poster
- Document Year:
- 2024
- Event:
- Categories:
- Log in to post comments
With neuroimaging data scientists have gained substantial information on the neuronal underpinning of intelligence. Yet how to integrate multimodal neuronal features effectively in relation to intelligence remains elusive. In this paper, we have developed a reference Canonical Correlation Analysis (RCCA) model that extracts latent, correlated multimodal features while enhancing correlation to a reference of interest. We applied RCCA to gray matter and white matter images from 7874 participants, and compared the derived features with those from Principle Components Analysis (PCA) and sparse CCA (SCCA), in terms of association with intelligence and prediction effectiveness using LASSO regression models. Using much fewer features RCCA achieves approximately equal r-squares than PCA and higher r-squares than SCCA. Our results indicate RCCA model can successfully extract features of interest. The top brain regions that contribute to intelligence include the cerebral tonsil, insula, lingual gyrus and middle occipital gyrus.