Documents
Research Manuscript
Multiscale Audio Spectrogram Transformer for Efficient Audio Classification
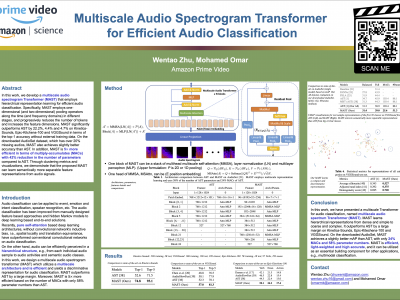
- Citation Author(s):
- Submitted by:
- Wentao Zhu
- Last updated:
- 19 May 2023 - 1:07pm
- Document Type:
- Research Manuscript
- Event:
- Categories:
- Log in to post comments
Audio event has a hierarchical architecture in both time and frequency and can be grouped together to construct more abstract semantic audio classes. In this work, we develop a multiscale audio spectrogram Transformer (MAST) that employs hierarchical representation learning for efficient audio classification. Specifically, MAST employs one-dimensional (and two-dimensional) pooling operators along the time (and frequency domains) in different stages, and progressively reduces the number of tokens and increases the feature dimensions. MAST significantly outperforms AST by 22.2%, 4.4% and 4.7% on Kinetics-Sounds, Epic-Kitchens-100 and VGGSound in terms of the top-1 accuracy without external training data. On the downloaded AudioSet dataset, which has over 20% missing audios, MAST also achieves slightly better accuracy than AST. In addition, MAST is 5x more efficient in terms of multiply-accumulates (MACs) with 42% reduction in the number of parameters compared to AST. Through clustering metrics and visualizations, we demonstrate that the proposed MAST can learn semantically more separable feature representations from audio signals.