Documents
Presentation Slides
MULTIVARIATE EMPIRICAL MODE DECOMPOSITION BASED SIGNAL ANALYSIS ANDEFFICIENT-STORAGE IN SMART GRID
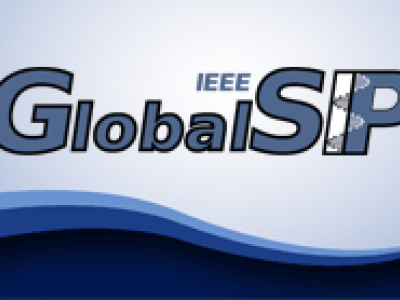
- Citation Author(s):
- Submitted by:
- Liu Liu
- Last updated:
- 5 December 2016 - 5:21pm
- Document Type:
- Presentation Slides
- Document Year:
- 2016
- Event:
- Presenters:
- Liu Liu
- Paper Code:
- 1429
- Categories:
- Log in to post comments
Wide-area-measurement systems (WAMSs) are used in smart grid systems to enable the efficient monitoring of grid dynamics. However, the overwhelming amount of data and the severe contamination from noise often impede the effective and efficient data analysis and storage of WAMS generated measurements. To solve this problem, we propose a novel framework that takes advantage of Multivariate Empirical Mode Decomposition (MEMD), a fully data-driven approach to analyzing non-stationary signals, dubbed MEMD based Signal Analysis (MSA). The frequency measurements are considered as a linear superposition of different oscillatory components and noise. The low-frequency components, corresponding to the long-term trend and inter-area oscillations, are grouped and compressed by MSA using the mean shift clustering algorithm. Whereas, higher-frequency components, mostly noise and potentially part of high-frequency inter-area oscillations, are analyzed using Hilbert spectral analysis and they are delineated by statistical behavior. By conducting experiments on both synthetic and real-world data, we show that the proposed framework can capture the characteristics, such as trends and inter-area oscillation, while reducing the data storage requirements.