Documents
Poster
MUSIC CHORD RECOGNITION BASED ON MIDI-TRAINED DEEP FEATURE AND BLSTM-CRF HYBIRD DECODING
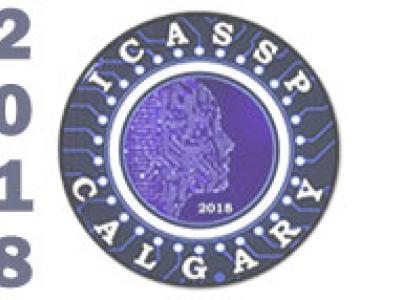
- Citation Author(s):
- Submitted by:
- Yiming Wu
- Last updated:
- 19 April 2018 - 10:25pm
- Document Type:
- Poster
- Document Year:
- 2018
- Event:
- Presenters:
- Yiming Wu
- Paper Code:
- 1753
- Categories:
- Log in to post comments
In this paper, we design a novel deep learning based hybrid system for automatic chord recognition. Currently, there is a bottleneck in the amount of enough annotated data for training robust acoustic models, as hand annotating time-synchronized chord labels requires professional musical skills and considerable labor. As a solution to this problem, we construct a large set of time synchronized MIDI-audio pairs, and use these data to train a Deep Residual Network (DRN) feature extractor, which can then estimate pitch class activations of real-world music audio recordings. Sequence classification and decoding are then performed with a trained Bidirectional LSTM and Conditional Random Fields (CRF) network. Experiments show that the proposed model is compatible for both regular major/minor triad chord classification and larger vocabulary chord recognition, the performance is good and no less than other state-of-the-art systems. The proposed system also achieved good evaluation score in MIREX 2017 Automatic Chord Estimation task.