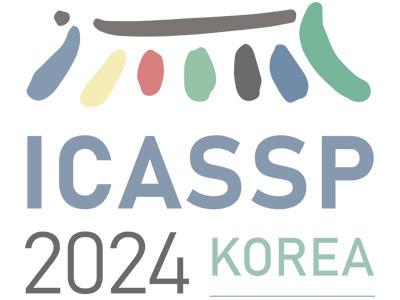
Music source separation (MSS) aims to separate a music recording into multiple musically distinct stems, such as vocals, bass, drums, and more. Recently, deep learning approaches such as convolutional neural networks (CNNs) and recurrent neural networks (RNNs) have been used, but the improvement is still limited. In this paper, we propose a novel frequency-domain approach based on a Band-Split RoPE Transformer (called BS-RoFormer).
- Categories:
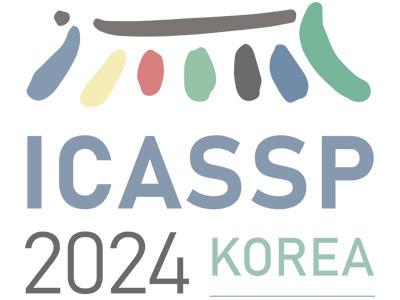
- Read more about MUSICLDM: ENHANCING NOVELTY IN TEXT-TO-MUSIC GENERATION USING BEAT-SYNCHRONOUS MIXUP STRATEGIES
- Log in to post comments
Diffusion models have shown promising results in cross-modal generation tasks, including text-to-image and text-to-audio generation. However, generating music, as a special type of audio, presents unique challenges due to limited availability of music data and sensitive issues related to copyright and plagiarism. In this paper, to tackle these challenges, we first construct a state-of-the-art text-to-music model, MusicLDM, that adapts Stable Diffusion and AudioLDM architectures to the music domain.
- Categories:
- Read more about PERFORMANCE CONDITIONING FOR DIFFUSION-BASED MULTI-INSTRUMENT MUSIC SYNTHESIS
- Log in to post comments
Generating multi-instrument music from symbolic music representations is an important task in Music Information Retrieval (MIR). A central but still largely unsolved problem in this context is musically and acoustically informed control in the generation process. As the main contribution of this work, we propose enhancing control of multi-instrument synthesis by conditioning a generative model on a specific performance and recording environment, thus allowing for better guidance of timbre and style.
- Categories:
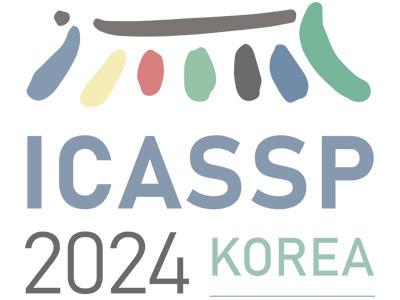
- Read more about THE USTC SYSTEM FOR CADENZA 2024 CHALLENGE
- Log in to post comments
This paper reports our submission to the ICASSP 2024 Cadenza Challenge, focusing on the non-causal system. The challenge aims to develop a signal processing system that enables personalized rebalancing of music to improve the listening experience for individuals with hearing loss when they listen to music via their hearing aids. The system is based on the Hybrid Demucs model. We fine-tuned the baseline model on the given dataset with a multi-target strategy and added a mixture of information in the downmixing stage.
- Categories:
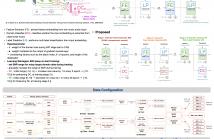
- Read more about MUSIC AUTO-TAGGING WITH ROBUST MUSIC REPRESENTATION LEARNED VIA DOMAIN ADVERSARIAL TRAINING
- Log in to post comments
Music auto-tagging is crucial for enhancing music discovery and recommendation. Existing models in Music Information Retrieval (MIR) struggle with real-world noise such as environmental and speech sounds in multimedia content. This study proposes a method inspired by speech-related tasks to enhance music auto-tagging performance in noisy settings. The approach integrates Domain Adversarial Training (DAT) into the music domain, enabling robust music representations that withstand noise.
- Categories:
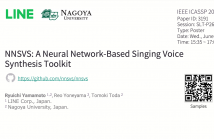
- Read more about NNSVS: A Neural Network-Based Singing Voice Synthesis Toolkit
- Log in to post comments
This paper describes the design of NNSVS, an open-source software for neural network-based singing voice synthesis research. NNSVS is inspired by Sinsy, an open-source pioneer in singing voice synthesis research, and provides many additional features such as multi-stream models, autoregressive fundamental frequency models, and neural vocoders. Furthermore, NNSVS provides extensive documentation and numerous scripts to build complete singing voice synthesis systems.
- Categories:
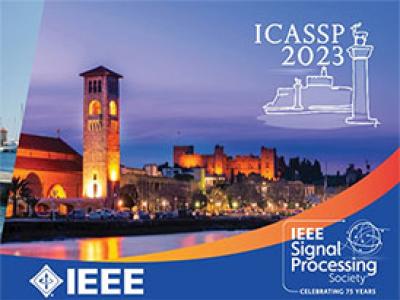
- Read more about A study of audio mixing methods for piano transcription in violin-piano ensembles
- Log in to post comments
- Categories:
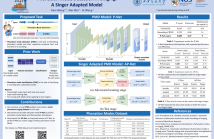
Phonation modes play a vital role in voice quality evaluation and vocal health diagnosis. Existing studies on phonation modes cover feature analysis and classification of vowels, which does not apply to real-life scenarios. In this paper, we define the phonation mode detection (PMD) problem, which entails the prediction of phonation mode labels as well as their onset and offset timestamps.
- Categories:
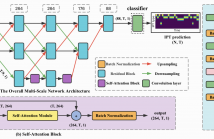
- Read more about Frame-Level Multi-Label Playing Technique Detection Using Multi-Scale Network and Self-Attention Mechanism
- Log in to post comments
Instrument playing technique (IPT) is a key element of musical presentation. However, most of the existing works for IPT detection only concern monophonic music signals, yet little has been done to detect IPTs in polyphonic instrumental solo pieces with overlapping IPTs or mixed IPTs. In this paper, we formulate it as a framelevel multi-label classification problem and apply it to Guzheng, a Chinese plucked string instrument. We create a new dataset, Guzheng Tech99, containing Guzheng recordings and onset, offset, pitch, IPT annotations of each note.
FRAME(1).pdf

- Categories:
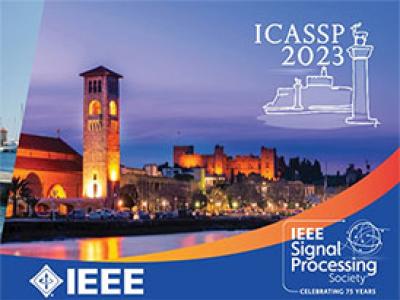
- Read more about IMPROVING MUSIC GENRE CLASSIFICATION FROM MULTI-MODAL PROPERTIES OF MUSIC AND GENRE CORRELATIONS PERSPECTIVE
- Log in to post comments
- Categories: