Documents
Poster
Poster
In-Network Linear Regression with Arbitrarily Split Data Matrices
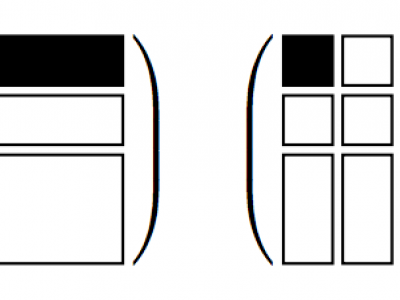
- Citation Author(s):
- Submitted by:
- Francois Cote
- Last updated:
- 5 December 2016 - 6:33pm
- Document Type:
- Poster
- Document Year:
- 2016
- Event:
- Presenters:
- François D. Côté
- Paper Code:
- RMN-P1.13
- Categories:
- Keywords:
- Log in to post comments
We address for the first time the question of how networked agents can collaboratively fit a Morozov-regularized linear model when each agent knows a summand of the regression data. This question generalizes previously studied data-splitting scenarios, which require that the data be partitioned among the agents. To answer the question, we introduce a class of network-structured problems, which contains the regularization problem, and by using the Douglas-Rachford splitting algorithm, we develop a distributed algorithm to solve these problems. We illustrate through simulations that our approach is an effective strategy for fully distributed linear regression.
Poster.pdf
