- Communication and Sensing aspects of Sensor Networks, Wireless and Ad-Hoc Networks
- Communication Systems and Applications
- MIMO Communications and Signal Processing
- Signal Transmission and Reception
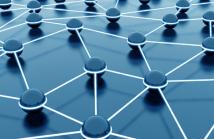
- Read more about OPTIMUM DECISION FUSION IN COGNITIVE WIRELESS SENSOR NETWORKS WITH UNKNOWN USERS LOCATION
- Log in to post comments
We consider a cooperative cognitive wireless network sce- nario where a primary wireless network is co-located with a cognitive (or secondary) network. In the considered scenario, the nodes of the secondary network make local binary de- cisions about the presence of a signal emitted by a primary node. Then, they transmit their decisions to a fusion center (FC). The final decision about the channel state is up to the FC by means of a proper fusion rule.
- Categories:
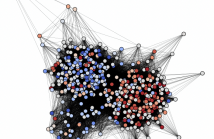
- Read more about Distributed Decision-Making for Community Structured Networks
- Log in to post comments
Traditional social learning frameworks consider environments with a homogeneous state where each agent receives observations conditioned on the same hypothesis. In this work, we study the distributed hypothesis testing problem for graphs with a community structure, assuming that each cluster receives data conditioned on some different true state. This situation arises in many scenarios, such as when sensors are spatially distributed, or when individuals in a social network have differing views or opinions.
- Categories:
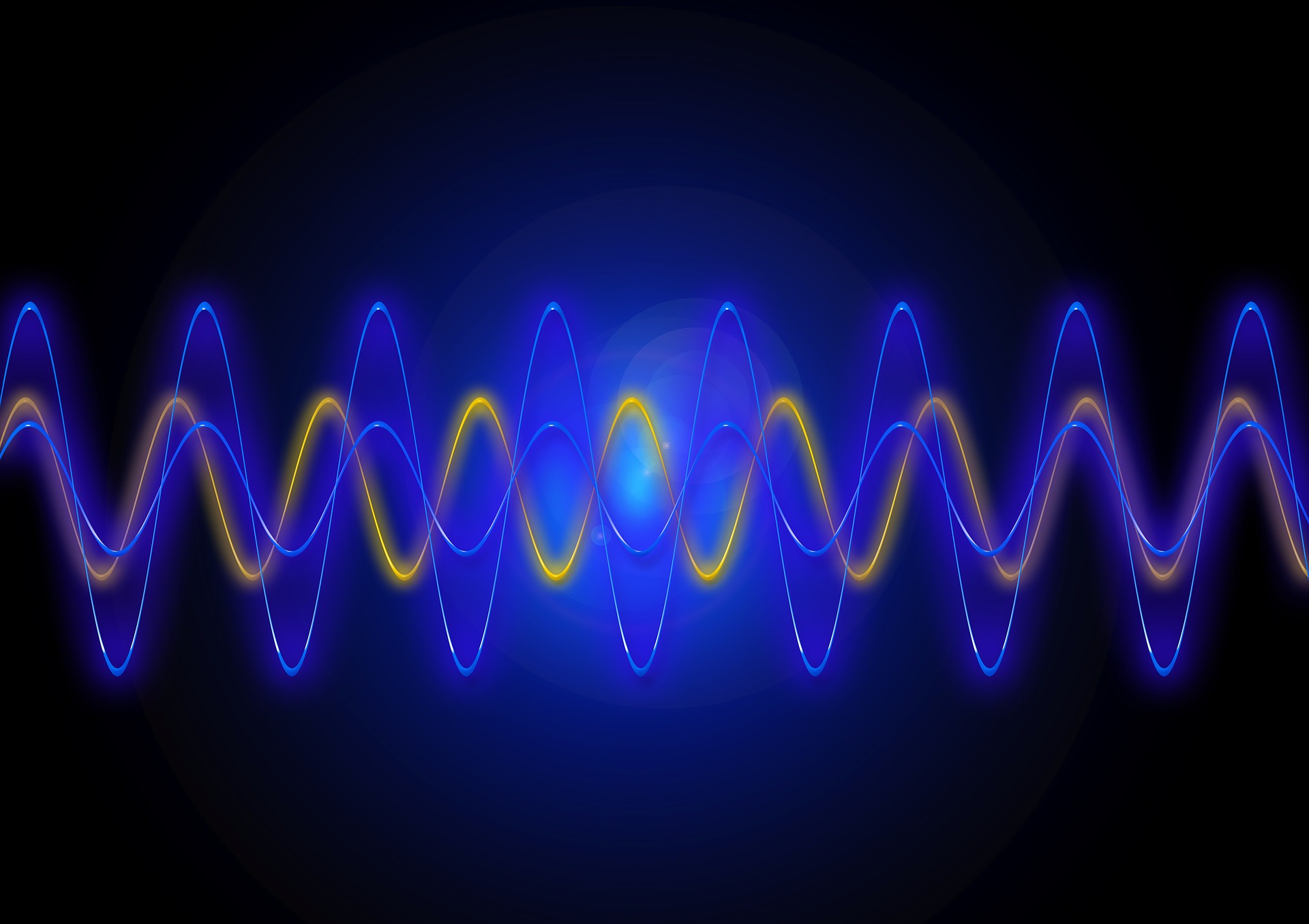
- Read more about Enhanced Channel Estimation in mm-Wave MIMO Systems Leveraging Integrated Communication and Sensing
- Log in to post comments
This paper tackles the challenge of wideband MIMO channel estimation within indoor millimeter-wave scenarios. Our proposed approach exploits the integrated sensing and communication paradigm, where sensing information aids in channel estimation. The key innovation consists of employing both spatial and temporal sensing modes to significantly reduce the number of required training pilots. Moreover, our algorithm addresses and corrects potential mismatches between sensing and communication modes, which can arise from differing sensing and communication propagation paths.
- Categories:
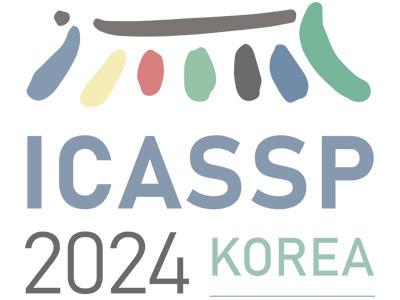
Reconfigurable intelligent surfaces (RISs) have been considered recently for target localization. While existing literature typically uses fixed RISs in the environment, mounting RISs on targets is a novel approach that can improve target visibility and positioning. This study derives the Cramér-Rao bound (CRB) for pose estimation (i.e., RIS position and orientation) under a generic wideband and near-field model. The theoretical findings show that a pose-dependent filtering phenomenon occurs, impacting the CRB, which is neglected under narrowband approximation.
- Categories:
- Read more about TOWARDS FASTER END-TO-END DATA TRANSMISSION OVER VOICE CHANNELS
- Log in to post comments
As new technologies spread, phone fraud crimes have become master strategies to steal money and personal identities. Inspired by website authentication, we propose an end-to-end data modem over voice channels that can transmit the caller’s digital certificate to the callee for verification. Without assistance from telephony providers, it is difficult to carry useful information over voice channels. For example, voice activity detection may quickly classify the encoded signals as nonspeech signals and reject the input waveform.
会议海报-终稿.pdf

- Categories:
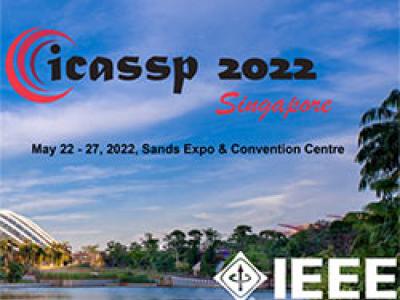
- Read more about Trade-offs in decentralized multi-antenna architectures: The WAX decomposition
- Log in to post comments
Current research on multi-antenna architectures is trending towards increasing the amount of antennas in the base stations (BSs) so as to increase the spectral efficiency. As a result, the interconnection bandwidth and computational complexity required to process the data using centralized architectures is becoming prohibitively high. Decentralized architectures can reduce these requirements by pre-processing the data before it arrives at a central processing unit (CPU).
- Categories:
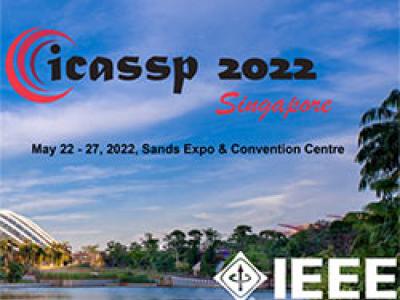
Cell-free massive multiple-input multiple-output (MIMO) consists of a large set of distributed access points (APs) serving a number of users. The APs can be far from each other, and they can also have a big number of antennas. Thus, decentralized architectures have to be considered so as to reduce the interconnection bandwidth to a central processing unit (CPU) and make the system scalable. On the other hand, the APs in a heterogeneous network might have limited processing capabilities and fully-decentralized processing may not be available.
- Categories:
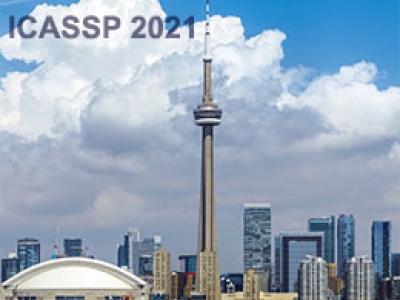
- Read more about Learning Bollobás-Riordan Graphs Under Partial Observability - Presentation Slides
- Log in to post comments
This work examines the problem of learning the topology of a network (graph learning) from the signals produced at a subset of the network nodes (partial observability). This challenging problem was recently tackled assuming that the topology is drawn according to an Erdős-Rényi model, for which it was shown that graph learning under partial observability is achievable, exploiting in particular homogeneity across nodes and independence across edges.
- Categories:
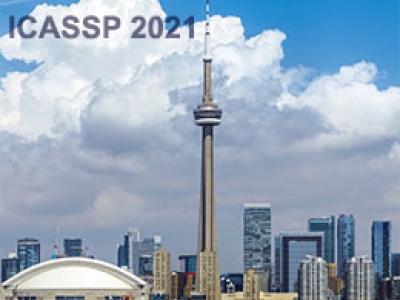
- Read more about Learning Bollobás-Riordan Graphs Under Partial Observability - Poster
- Log in to post comments
This work examines the problem of learning the topology of a network (graph learning) from the signals produced at a subset of the network nodes (partial observability). This challenging problem was recently tackled assuming that the topology is drawn according to an Erdős-Rényi model, for which it was shown that graph learning under partial observability is achievable, exploiting in particular homogeneity across nodes and independence across edges.
- Categories:
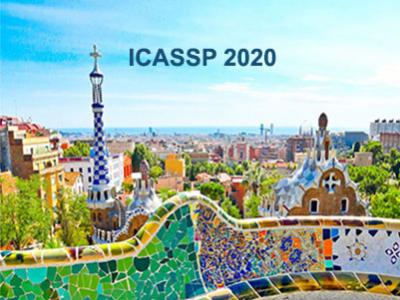
- Read more about Sparse Beamspace Equalization for Massive MU-MIMO mmWave Systems
- Log in to post comments
- Categories: