Documents
Poster
A NEURAL NETWORK BASED RANKING FRAMEWORK TO IMPROVE ASR WITH NLU RELATED KNOWLEDGE DEPLOYED
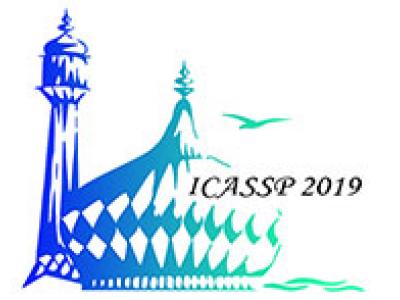
- Citation Author(s):
- Submitted by:
- Zhengyu Zhou
- Last updated:
- 7 May 2019 - 7:57pm
- Document Type:
- Poster
- Document Year:
- 2019
- Event:
- Presenters:
- Zhengyu Zhou
- Paper Code:
- SLP-P11.6
- Categories:
- Keywords:
- Log in to post comments
This work proposes a new neural network framework to simultaneously rank multiple hypotheses generated by one or more automatic speech recognition (ASR) engines for a speech utterance. Features fed in the framework not only include those calculated from the ASR information, but also involve natural language understanding (NLU) related features, such as trigger features capturing long-distance constraints between word/slot pairs and BLSTM features representing intent-sensitive sentence embedding. The framework predicts the ranking result of the input hypotheses, outputting the top-ranked hypothesis as the new ASR result together with its slot filling and intention detection results. We conduct the experiments on an in-car infotainment corpus and the ATIS (Airline Travel Information Systems) corpus, for which hypotheses are generated by different types of engines and a single engine, respectively. The experimental results achieved are encouraging on both data corpora (e.g., 21.9% relative reduction in word error rate over state-of-the-art Google cloud ASR performance on the ATIS testing data), proving the effectiveness of the proposed ranking framework.