Documents
Presentation Slides
Presentation Slides
Neural Ordinary differential equations with Trainable solvers
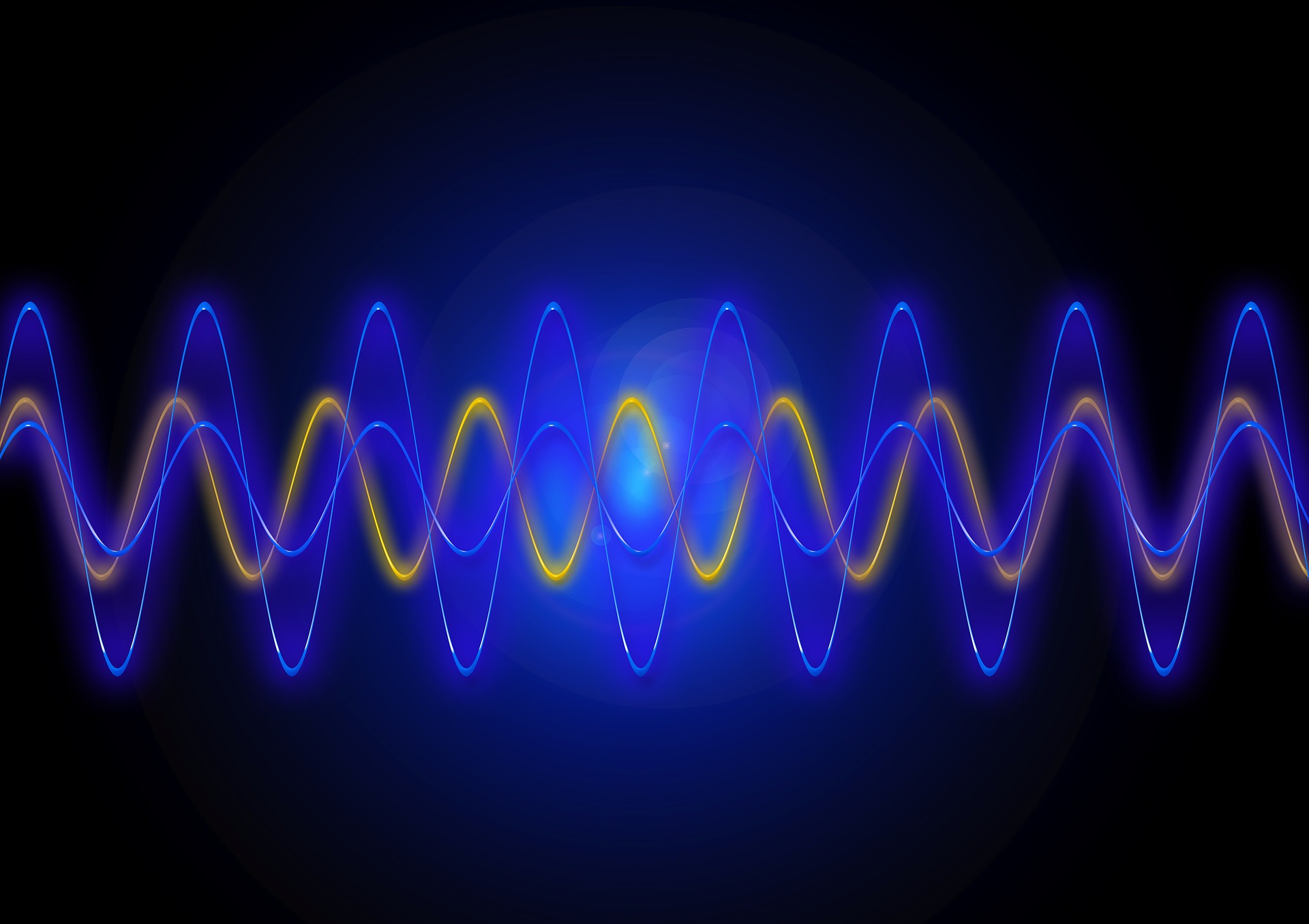
- DOI:
- 10.60864/925v-1g39
- Citation Author(s):
- Submitted by:
- Said Ouala
- Last updated:
- 6 June 2024 - 10:50am
- Document Type:
- Presentation Slides
- Categories:
- Log in to post comments
When considering the data-driven identification of non-linear differential equations, the choice of the integration scheme to use is far from being trivial and may dramatically impact the identification problem. In this work, we discuss this aspect and propose a novel architecture that jointly learns Neural Ordinary Differential Equations (NODEs) as well as the corresponding integration schemes that would minimize the forecast of a given sequence of observations. We demonstrate its relevance with numerical experiments on non-linear dynamics, including chaotic systems.
Comments
Presentation Slides
Presentation Slides