Documents
Poster
Noise-Aware Speech Separation with Contrastive Learning
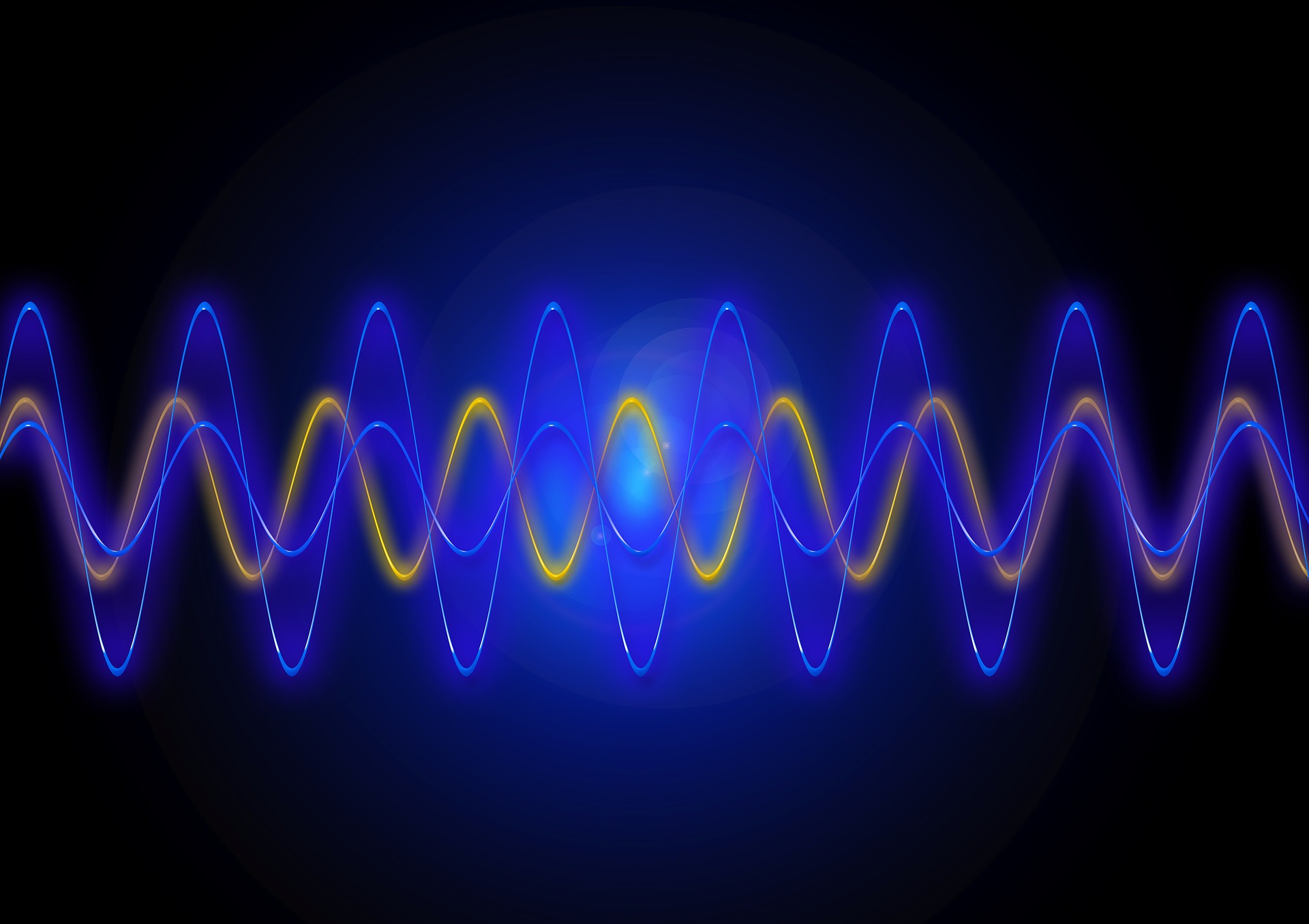
- DOI:
- 10.60864/qdr7-gc47
- Citation Author(s):
- Submitted by:
- zizheng zhang
- Last updated:
- 6 June 2024 - 10:28am
- Document Type:
- Poster
- Categories:
- Log in to post comments
Recently, speech separation (SS) task has achieved remarkable progress driven by deep learning technique. However, it is still challenging to separate target speech from noisy mixture, as the neural model is vulnerable to assign background noise to each speaker. In this paper, we propose a noise-aware SS (NASS) method, which aims to improve the speech quality for separated signals under noisy conditions. Specifically, NASS views background noise as an additional output and predicts it along with other speakers in a mask-based manner. To effectively denoise, we introduce patch-wise contrastive learning (PCL) between noise and speaker representations from the decoder input and encoder output. PCL loss aims to minimize the mutual information between predicted noise and other speakers at multiple-patch level to suppress the noise information in separated signals. Experimental results show that NASS achieves 1 to 2dB SI-SNRi or SDRi over DPRNN and Sepformer on WHAM! and LibriMix noisy datasets, with less than 0.1M parameter increase.
poster.pdf

Comments
github repo: https://github
github repo: https://github.com/TzuchengChang/NASS