Documents
Poster
A Novel Method for Human Bias Correction of Continuous-time Annotations
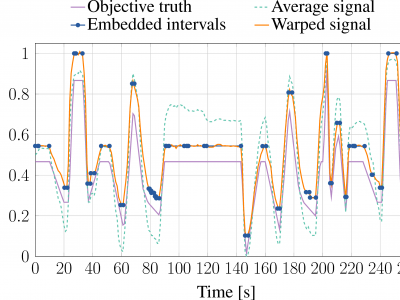
- Citation Author(s):
- Submitted by:
- Brandon Booth
- Last updated:
- 13 April 2018 - 2:06pm
- Document Type:
- Poster
- Document Year:
- 2018
- Event:
- Presenters:
- Brandon M Booth
- Paper Code:
- 4133
- Categories:
- Log in to post comments
Human annotations are of integral value in human behavior studies and in particular for the generation of ground truth for behavior prediction using various machine learning methods. These often subjective human annotations are especially required for studies involving measuring and predicting hidden mental states (e.g. emotions) that cannot effectively be measured or assessed by other means. Human annotations are noisy and prone to the influence of several factors including personal bias, task ambiguity, environmental distractions, and health state. We propose a novel method for fusion of continuous real-time human annotations to generate accurate ground truth estimates. We introduce a signal warping method that uses additional comparative rank-based information about specific subsets of the annotations to correct for specific types of human annotation artifacts. This approach is validated using a mechanically simple but perceptually demanding psycho-physical annotation experiment where objective truth labels are known. Our method yields ground truth estimates that are in better agreement with the objective truth than state-of-the-art approaches.