Documents
Presentation Slides
Paper_8423_Xin_Zhu
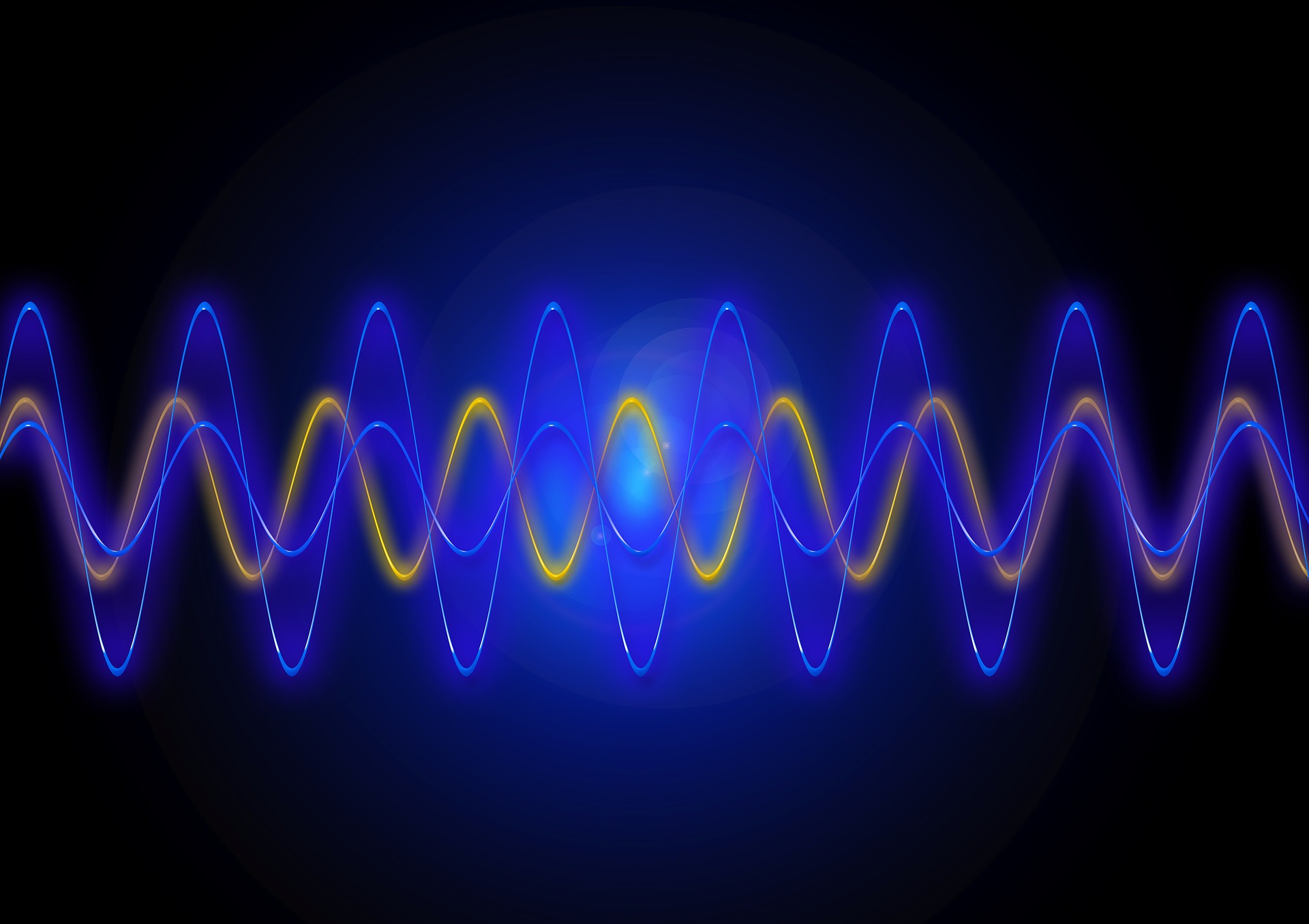
- DOI:
- 10.60864/zqn3-5c02
- Citation Author(s):
- Submitted by:
- Xin Zhu
- Last updated:
- 6 June 2024 - 10:24am
- Document Type:
- Presentation Slides
- Document Year:
- 2024
- Presenters:
- Xin Zhu
- Paper Code:
- 8423
- Categories:
- Log in to post comments
Electroencephalogram (EEG) data compression is necessary for wireless recording applications to reduce the amount of data that needs to be transmitted. In this paper, an asymmetrical sparse autoencoder with a discrete cosine transform (DCT) layer is proposed to compress EEG signals. The encoder module of the autoencoder has a combination of a fully connected linear layer and the DCT layer to reduce redundant data using hard-thresholding nonlinearity. Furthermore, the DCT layer includes trainable hard-thresholding parameters and scaling layers to give emphasis or de-emphasis on individual DCT coefficients. Finally, the one-by-one convolutional layer generates the latent space. The sparsity penalty-based cost function is employed to keep the feature map as sparse as possible in the latent space. The latent space data is transmitted to the receiver.
The decoder module of the autoencoder is designed using the inverse DCT and two fully connected linear layers to improve the accuracy of data reconstruction. In comparison to other state-of-the-art methods, the proposed method significantly improves the average quality score in various data compression experiments.