Documents
Presentation Slides
PerceptNet: A Human Visual System Inspired Neural Network for Estimating Perceptual Distance
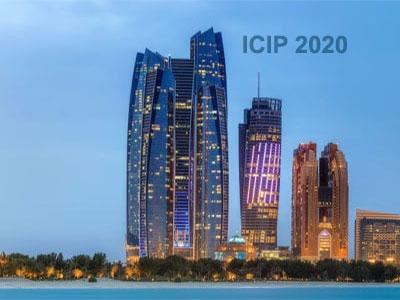
- Citation Author(s):
- Submitted by:
- Alexander Hepburn
- Last updated:
- 2 November 2020 - 2:26pm
- Document Type:
- Presentation Slides
- Document Year:
- 2020
- Event:
- Presenters:
- Alexander Hepburn
- Categories:
- Keywords:
- Log in to post comments
Traditionally, the vision community has devised algorithms to estimate the distance between an original image and images that have been subject to perturbations. Inspiration was usually taken from the human visual perceptual system and how the system processes different perturbations in order to replicate to what extent it determines our ability to judge image quality. While recent works have presented deep neural networks trained to predict human perceptual quality, very few borrow any intuitions from the human visual system. To address this, we present PerceptNet, a convolutional neural network where the architecture has been chosen to reflect the structure and various stages in the human visual system. We evaluate PerceptNet on various traditional perception datasets and note strong performance on a number of them as compared with traditional image quality metrics. We also show that including a nonlinearity inspired by the human visual system in classical deep neural networks architectures can increase their ability to judge perceptual similarity. Compared to similar deep learning methods, the performance is similar, although our network has a number of parameters that is several orders of magnitude less.