Documents
supplementar material
PPREDICTING UNCANNY PERCEPTION IN VIRTUAL HUMANS FACES THROUGH IMAGE FEATURES
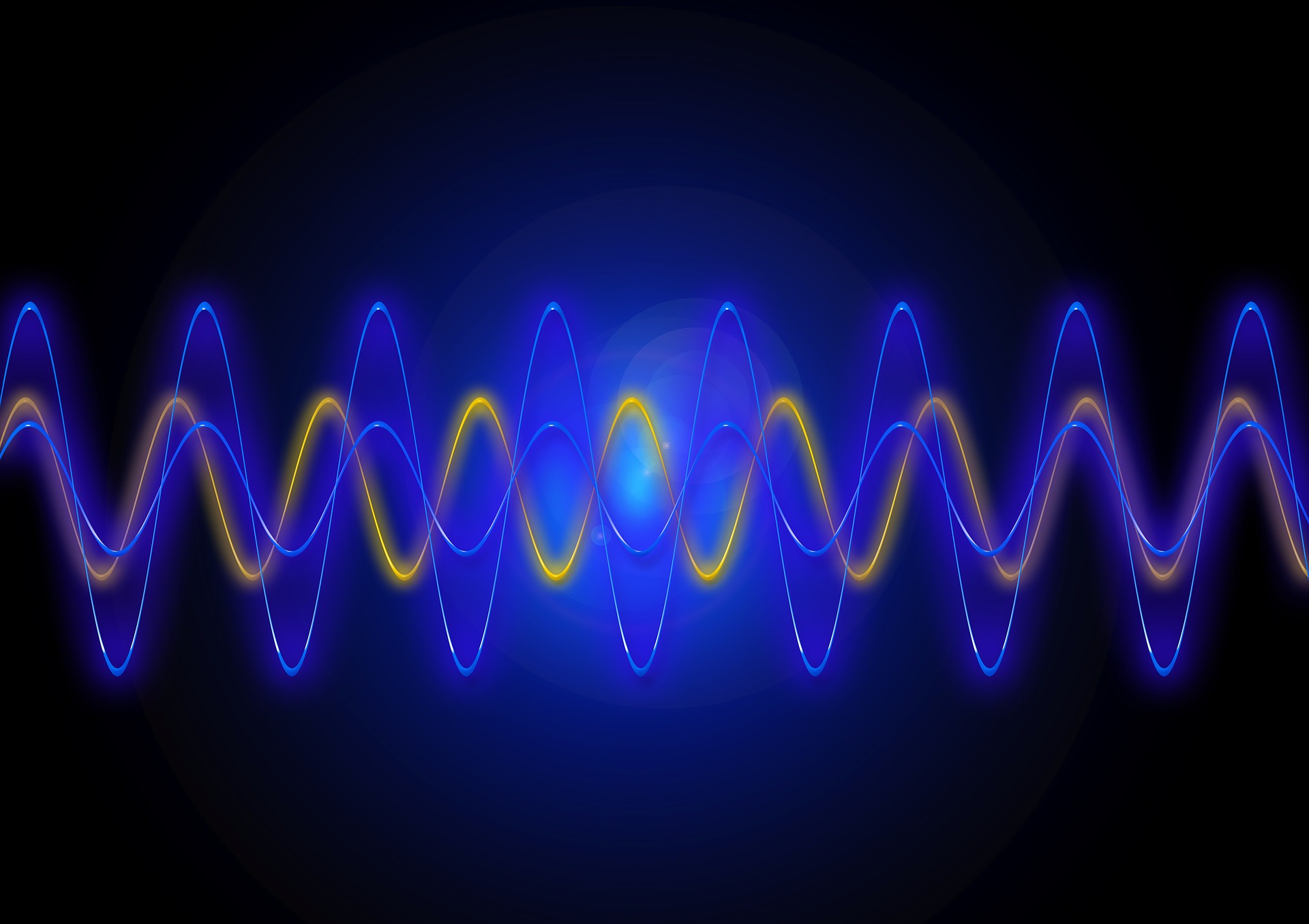
- Citation Author(s):
- Submitted by:
- Greice Dal Molin
- Last updated:
- 19 January 2025 - 5:27pm
- Document Type:
- supplementar material
- Categories:
- Log in to post comments
The perception of strangeness or discomfort in virtual humans, as described by the Uncanny Valley (UV) theory, plays a critical role in shaping users’ interactions with technology. Understanding this phenomenon is essential to reduce its impact during the design and animation of virtual humans. While numerous studies have explored the subjective dimensions of the UV effect, focusing on human perception, our research proposes a novel methodology to investigate this phenomenon by correlating objective image character istics of virtual humans with users’ subjective perceptions of discomfort. We introduce VHFACE, a dataset contain ing 8,099 images of 40 characters from video games and computer-generated films, annotated with ground truth regarding perceived strangeness. A variety of image features, including HOG, Hu moments, Action Units (Ekman), entropy, and others, were analyzed using four machine learning techniques. Additionally, we evaluated the predictive accuracy of Convolutional Neural Networks (CNNs). Although CNNs did not outperform other methods, our results demonstrate that ensemble approaches like Voting Classifier and Voting Regressor achieved higher precision, with 67.5% and 80% accuracy rates, respectively. These findings reflect the inherent subjectivity of human perception, which is influ-
enced by numerous factors, underscoring the importance of combining multiple models for robust predictions.