Documents
Presentation Slides
QA4QG: USING QUESTION ANSWERING TO CONSTRAIN MULTI-HOP QUESTION GENERATION
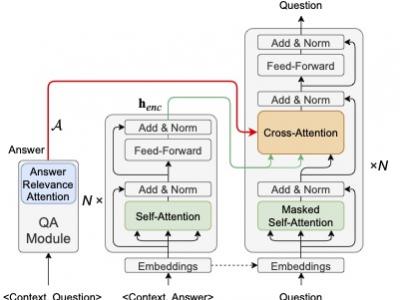
- Citation Author(s):
- Submitted by:
- Dan Su
- Last updated:
- 11 May 2022 - 6:32am
- Document Type:
- Presentation Slides
- Document Year:
- 2022
- Event:
- Presenters:
- Dan SU
- Categories:
- Log in to post comments
Multi-hop question generation (MQG) aims to generate complex questions which require reasoning over multiple pieces of information of the input passage. Most existing work on MQG has focused on exploring graph-based networks to equip the traditional Sequence-to-sequence framework with reasoning ability. However, these models do not take full advantage of the constraint between questions and answers. Furthermore, studies on multi-hop question answering (QA) suggest that Transformers can replace the graph structure for multi-hop reasoning. Therefore, in this work, we propose a novel framework, QA4QG, a QA-augmented BART-based framework for MQG. It augments the standard BART model with an additional multi-hop QA module to further constrain the generated question. Our results on the HotpotQA dataset show that QA4QG outperforms all state-of-the-art models, with an increase of 8 BLEU-4 and 8 ROUGE points compared to the best results previously reported. Our work suggests the advantage of introducing pre-trained language models and QA module for the MQG task.