Documents
Presentation Slides
Reconstruction-free deep convolutional neural networks for partially observed images
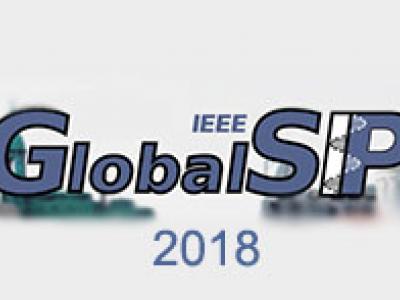
- Citation Author(s):
- Submitted by:
- Luoluo Liu
- Last updated:
- 26 November 2018 - 8:14pm
- Document Type:
- Presentation Slides
- Document Year:
- 2018
- Event:
- Categories:
- Log in to post comments
Conventional image discrimination tasks are performed on fully observed images. In challenging real imaging scenarios, where sensing systems are energy demanding or need to operate with limited bandwidth and exposure-time budgets, or defective pixels, where the data collected often suffers from missing information, and this makes the task extremely hard. In this paper, we leverage Convolutional Neural Networks (CNNs) to extract information from partially observed images. While pre-trained CNNs fail significantly even with such a small percentage of the input missing, our proposed framework demonstrates the ability to overcome it after training on fully-observed and partially-observed images at a few observation ratios. We demonstrate that our method is indeed reconstruction-free, retraining-free and generalizable to previously untrained-on observation ratios and it remains effective in two different visual tasks – image classification and object detection. Our framework performs well even for test images with only 10% of pixels available and outperforms the reconstruct-then-classify pipeline in these challenging scenarios for small observation fractions.