Documents
Poster
Rich Punctuations Prediction Using Large-scale Deep Learning
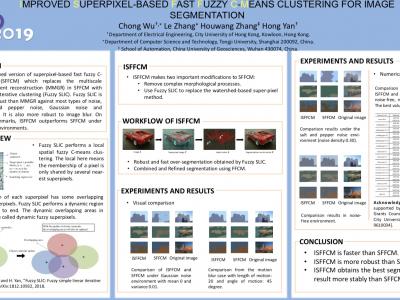
- Citation Author(s):
- Submitted by:
- Wu Xueyang
- Last updated:
- 14 October 2016 - 2:50am
- Document Type:
- Poster
- Document Year:
- 2016
- Event:
- Presenters:
- Xueyang Wu
- Paper Code:
- ISCSLP1601
- Categories:
- Log in to post comments
Punctuation plays an important role in language processing. However, automatic speech recognition systems only output plain word sequences. It is then of interest to predict punctuations on plain word sequences. Previous works have focused on using lexical features or prosodic cues captured from small corpus to predict simple punctuations. Compared with simple punctuations, rich punctuations provide more meaningful
information and are more difficult to predict. In this paper, a multi-view LSTM model is proposed to predict rich punctuations on large-scale corpora. In particular, predictions on both in-domain and out-of-domain datasets are investigated. Experiments showed that LSTM can significantly outperform the traditional CRF-based model. Moreover, large-scale corpora are proved to bring large progress, and introducing POS tags and Chunking information in a multi-view structure improves performance of LSTM model on small corpus.