Documents
Poster
ROBUST FEATURE CLUSTERING FOR UNSUPERVISED SPEECH ACTIVITY DETECTION
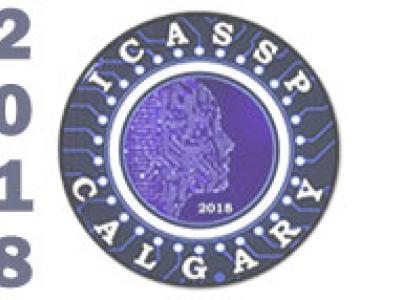
- Citation Author(s):
- Submitted by:
- Harishchandra Dubey
- Last updated:
- 19 April 2018 - 10:02pm
- Document Type:
- Poster
- Document Year:
- 2018
- Event:
- Presenters:
- Harishchandra Dubey
- Paper Code:
- 1504
- Keywords:
- Log in to post comments
In certain applications such as zero-resource speech processing
or very-low resource speech-language systems, it might
not be feasible to collect speech activity detection (SAD) annotations.
However, the state-of-the-art supervised SAD techniques
based on neural networks or other machine learning
methods require annotated training data matched to the target
domain. This paper establish a clustering approach for fully
unsupervised SAD useful for cases where SAD annotations
are not available. The proposed approach leverages Hartigan
dip test in a recursive strategy for segmenting the feature
space into prominent modes. Statistical dip is invariant
to distortions that lends robustness to the proposed method.
We evaluate the method on NIST OpenSAD 2015 and NIST
OpenSAT 2017 public safety communications data. The results
showed the superiority of proposed approach over the
two-component GMM baseline.