Documents
Poster
Poster
ROBUST ONLINE MATRIX COMPLETION WITH GAUSSIAN MIXTURE MODEL
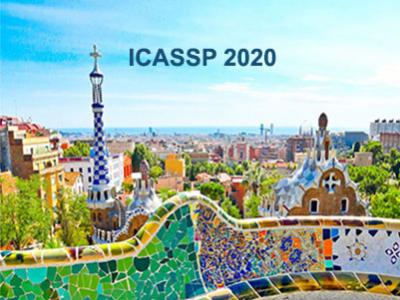
- Citation Author(s):
- Submitted by:
- spring liu
- Last updated:
- 13 May 2020 - 11:11pm
- Document Type:
- Poster
- Document Year:
- 2020
- Event:
- Presenters:
- Chunsheng Liu
- Paper Code:
- MLSP-P1.2
- Categories:
- Log in to post comments
In this paper, we study the problem of online matrix completion (MC) aiming to achieve robustness to the variations in both low-rank subspace and noises. In contrast to existing methods, we progressively fit a specific Gaussian Mixture Model (GMM) for noises at each time slot, which ensures the adaptiveness of the model to dynamic complex noises under real application scenarios. Consequently, we formalize the online MC into an optimization problem based on the GMM regularizer. In particular, embedding the EM framework, we proposed a fast and memory-efficient online MC algorithm to solve the resulting optimization problem. The performance of the proposed method is substantiated on both synthetic and real-world data sets.
ICASSP.pdf
