Documents
Poster
SELF-PACED LEAST SQUARE SEMI-COUPLED DICTIONARY LEARNING FOR PERSON RE-IDENTIFICATION
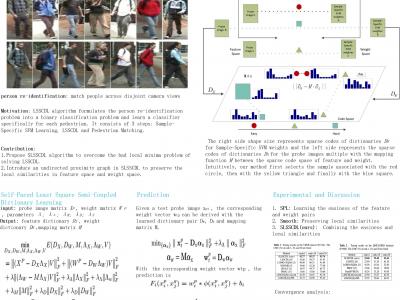
- Citation Author(s):
- Submitted by:
- Wei Xu
- Last updated:
- 7 September 2017 - 11:10am
- Document Type:
- Poster
- Document Year:
- 2017
- Event:
- Presenters:
- Wei Xu
- Paper Code:
- #2789
- Categories:
- Log in to post comments
Person re-identification aims to match people across disjoint camera views. It has been reported that Least Square
Semi-Coupled Dictionary Learning (LSSCDL) based samplespecific SVM learning framework has obtained the state of
the art performance. However, the objective function of the LSSCDL, the algorithm of learning the pairs (feature, weight)
dictionaries and the mapping function between feature space and weight space, is non-convex, which usually result in
suboptimal solutions with the bad local minima of the objective function. To tackle with this constraint, we present
Self-Paced Least Square Semi-Coupled Dictionary Learning (SLSSCDL) algorithm, which is inspired by previous
works on self-paced learning, a framework able to improve the accuracy of conventional learning models by presenting
the training data in a meaningful order to get a better local minima, i.e. easy samples are provided first. In addition, a
graph based regularization term is also introduced to preserve the local similarities in each space. Experimental results
show that SLSSCDL gains competitive performance on two challenging datasets.