Documents
Poster
Self-paced mixture of t distribution model
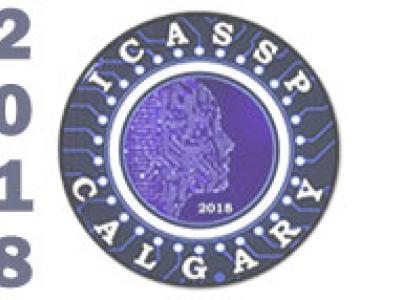
- Citation Author(s):
- Submitted by:
- Yang Zhang
- Last updated:
- 27 May 2018 - 10:23pm
- Document Type:
- Poster
- Document Year:
- 2018
- Event:
- Paper Code:
- 18001
- Categories:
- Keywords:
- Log in to post comments
Gaussian mixture model (GMM) is a powerful probabilistic model for representing the probability distribution of observations in the population. However, the fitness of Gaussian mixture model can be significantly degraded when the data contain a certain amount of outliers. Although there are certain variants of GMM (e.g., mixture of Laplace, mixture of t distribution) attempting to handle outliers, none of them can sufficiently mitigate the effect of outliers if the outliers are far from the centroids. Aiming to remove the effect of outliers further, this paper introduces a Self-Paced Learning mechanism into mixture of t distribution, which leads to Self-Paced Mixture of t distribution model (SPTMM). We derive an Expectation-Maximization based algorithm to train SPTMM and show SPTMM is able to screen the outliers. To demonstrate the effectiveness of SPTMM, we apply the model to density estimation and clustering. Finally, the results indicate that SPTMM outperforms other methods, especially on the data with outliers.