Documents
Presentation Slides
A SELF-SUPERVISED LEARNING APPROACH FOR DETECTING NON-PSYCHOTIC RELAPSES USING WEARABLE-BASED DIGITAL PHENOTYPING
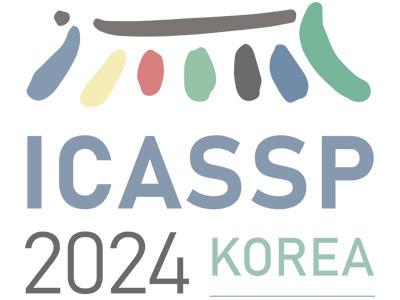
- DOI:
- 10.60864/bsef-1w91
- Citation Author(s):
- Submitted by:
- Theodoros Giann...
- Last updated:
- 15 April 2024 - 12:54pm
- Document Type:
- Presentation Slides
- Document Year:
- 2024
- Event:
- Presenters:
- Sofia Eleftheriou
- Paper Code:
- ICASSP2024-11921
- Categories:
- Log in to post comments
We present MagCIL's approach for the 1st track of the "2nd e-Prevention challenge: Psychotic and Non-Psychotic Relapse Detection using Wearable-Based Digital Phenotyping". First we present our approach for preprocessing and extracting features from the wearable's raw data. We then propose a Transformer model for learning self-supervised representations from augmented features, trained on data from non-relapse days from each of the 9 patients of the challenge. We adopt two unsupervised methods for detecting relapse days as outliers. A separate unsupervised model is tuned for each patient using the validation data of the challenge. Our method ranked second with ROC_AUC=0.651 and PR_AUC=0.642 on the final test dataset of the challenge. The respective code is available at this repository: https://github.com/magcil/e-prevention-challenge.