Documents
Presentation Slides
SELFISH LEARNING: LEVERAGING THE GREED IN SOCIAL LEARNING
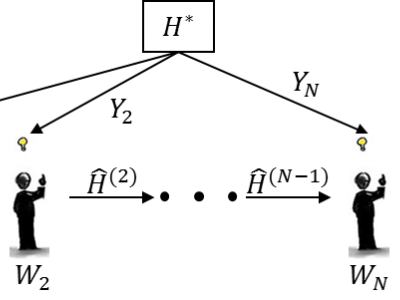
- Citation Author(s):
- Submitted by:
- Ravi Kiran Raman
- Last updated:
- 18 April 2018 - 1:02pm
- Document Type:
- Presentation Slides
- Document Year:
- 2018
- Event:
- Presenters:
- Ravi Kiran Raman
- Paper Code:
- MLSP-L4.1
- Categories:
- Log in to post comments
We introduce a sequential Bayesian binary hypothesis testing problem under social learning, termed selfish learning, where agents work to maximize their individual rewards. In particular, each agent receives a private signal and is aware of decisions made by earlier-acting agents. Beside inferring the underlying hypothesis, agents also decide whether to stop and declare, or pass the inference to the next agent. The employer rewards only correct responses and the reward per worker decreases with the number of employees used for decision making. We characterize decision regions of agents in the infinite and finite horizon. In particular, we show that the decision boundaries in the infinite horizon are the solutions to a Markov Decision Process with discounted costs, and can be solved using value iteration. In the finite horizon, we show that team performance is enhanced upon appropriate incentivization when compared to sequential social learning.