Documents
Poster
Semi-Supervised Transfer Learning for Convolutional Neural Networks for Glaucoma Detection
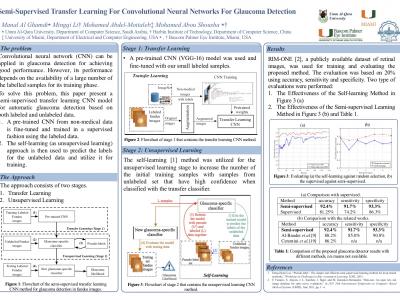
- Citation Author(s):
- Submitted by:
- Manal AlGhamdi
- Last updated:
- 9 May 2019 - 2:27pm
- Document Type:
- Poster
- Document Year:
- 2019
- Event:
- Presenters:
- Manal AlGhamdi, Mingqi Li
- Paper Code:
- ICASSP19005
- Categories:
- Log in to post comments
Convolutional neural network (CNN) can be applied in glaucoma detection for achieving good performance.
However, its performance depends on the availability of a large number of the labelled samples for its training phase.
To solve this problem, this paper present a semi-supervised transfer learning CNN model for automatic glaucoma detection based on both labeled and unlabeled data.
First, a pre-trained CNN from non-medical data is fine-tuned and trained in a supervised fashion using the labeled data.
The self-learning approach is then used to predict the labels for the unlabeled data and utilize it for training.
The experimental results on the RIM-ONE database demonstrate the effectiveness of the proposed algorithm despite the lack of initial labeled samples.