Documents
Poster
SINGLE DEPTH IMAGE SUPER-RESOLUTION USING CONVOLUTIONAL NEURAL NETWORKS
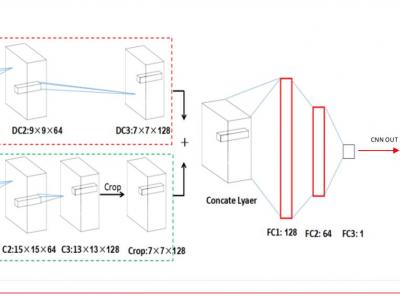
- Citation Author(s):
- Submitted by:
- Baoliang Chen
- Last updated:
- 12 April 2018 - 11:53pm
- Document Type:
- Poster
- Document Year:
- 2018
- Event:
- Presenters:
- Baoliang Chen
- Paper Code:
- ICASSP18001
- Categories:
- Log in to post comments
In this paper, a novel framework for the single depth image superresolution is proposed. In our framework, we first extract a low-quality edge map from an interpolated depth map.Then we transform the low-quality edge map to a high quality one by our trained deep convolution neural network (CNN) with two-step postprocessing. Guided by the high-quality edge map, we finally utilize a total variation (TV) based model to upsample the initial depth map. The high quality edge-based guidance not only helps avoiding artifacts introduced by direct texture prediction, but also reduces jagged artifacts and preserves the sharp edges. Experimental results demonstrate the effectiveness of our method both qualitatively and quantitatively compared with the state-of-the-art methods.