Documents
Poster
SINGLE DEPTH IMAGE SUPER-RESOLUTION USING CONVOLUTIONAL NEURAL NETWORKS
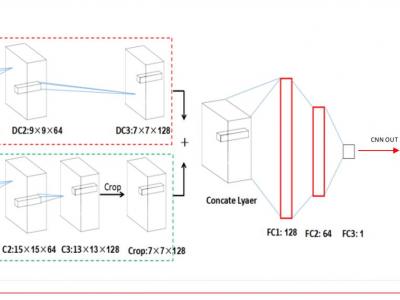
- Citation Author(s):
- Submitted by:
- Baoliang Chen
- Last updated:
- 12 April 2018 - 11:53pm
- Document Type:
- Poster
- Document Year:
- 2018
- Event:
- Presenters:
- Baoliang Chen
- Paper Code:
- 1979
- Categories:
- Log in to post comments
In this paper, we propose single depth image super-resolution using convolutional neural networks (CNN). We adopt CNN to acquire a high-quality edge map from the input low-resolution (LR) depth image. We use the high-quality edge map as the weight of the regularization term in a total variation (TV) model for super-resolution. First, we interpolate the LR depth image using bicubic interpolation and extract its low-quality edge map. Then, we get the high-quality edge map from the low-quality one using CNN. Since the CNN output often contains broken edges and holes, we refine it using the low-quality edge map. Guided by the high-quality edge map, we upsample the input LR depth image in the TV model. The edge-based guidance in TV effectively removes noise in depth while minimizing jagged artifacts and preserving sharp edges. Various experiments on the Middlebury stereo dataset and Laser Scan dataset demonstrate the superiority of the proposed method over state-of-the-arts in both qualitative and quantitative measurements.