Documents
Presentation Slides
Slides for CAGEN
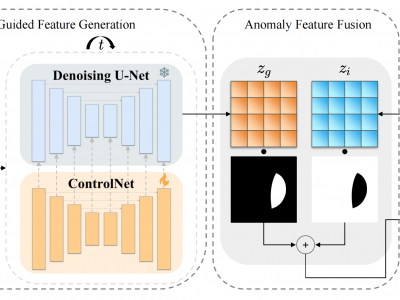
- DOI:
- 10.60864/g7bx-fm90
- Citation Author(s):
- Submitted by:
- Bolin Jiang
- Last updated:
- 15 April 2024 - 2:37pm
- Document Type:
- Presentation Slides
- Categories:
- Log in to post comments
Data augmentation has been widely applied in anomaly detection, which generates synthetic anomalous data for training. However, most existing anomaly augmentation methods focus on image-level cut-and-paste techniques, resulting in less realistic synthetic results, and are restricted to a few predefined patterns. In this paper, we propose our Controllable Anomaly Generator (CAGen) for anomaly data augmentation, which can generate high-quality images, and be flexibly controlled with text prompts. Specifically, our method finetunes a ControlNet model by using binary masks and textual prompts to control the spatial localization and style of generated anomalies. To further augment the resemblance between the generated features and normal samples, we propose a fusion method that integrates the generated anomalous features with the features of normal samples. Experiments on standard anomaly detection benchmarks show that the proposed data augmentation method significantly leads to a 0.4/3.1 improvement in the AUROC/AP metric.
ICASSP.pptx
