Documents
Presentation Slides
Sparse Support Recovery via Covariance Estimation
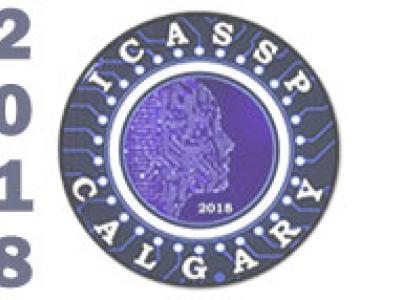
- Citation Author(s):
- Submitted by:
- Lekshmi Ramesh
- Last updated:
- 13 April 2018 - 4:06am
- Document Type:
- Presentation Slides
- Document Year:
- 2018
- Event:
- Presenters:
- Chandra R. Murthy
- Paper Code:
- SS-L3.6
- Categories:
- Log in to post comments
We consider the problem of recovering the common support of a set of
$k$-sparse signals $\{\mathbf{x}_{i}\}_{i=1}^{L}$ from noisy linear
underdetermined measurements of the form
$\{{\Phi} \mathbf{x}_{i}+\mathbf{w}_{i}\}_{i=1}^{L}$ where
${\Phi}\in\rr^{m\times N}$ $(m<N)$ is the sensing matrix and
$\mathbf{w}_{i}$ is the additive noise. We employ a Bayesian setup where we impose a Gaussian prior with zero mean and a
common diagonal covariance matrix $\mathbf{\Gamma}$ across all
$\mathbf{x}_{i},$ and formulate the support recovery problem as one of
covariance estimation.
We develop an algorithm to find the
approximate maximum-likelihood estimate of $\mathbf{\Gamma}$ using a
modified reweighted minimization procedure. Empirically, we
find that the proposed algorithm succeeds in exactly recovering
the common support with high probability in the $k<m$ regime with $L$
of the order of $m$ and in the $k\ge m$ regime with larger $L$. The key advantage of the proposed algorithm is that its complexity is independent of $L$, unlike existing sparse support recovery algorithms.
icassp_v3.pdf
