Documents
Supplementary Materials
STENCIL: Subject-Driven Generation with Context Guidance
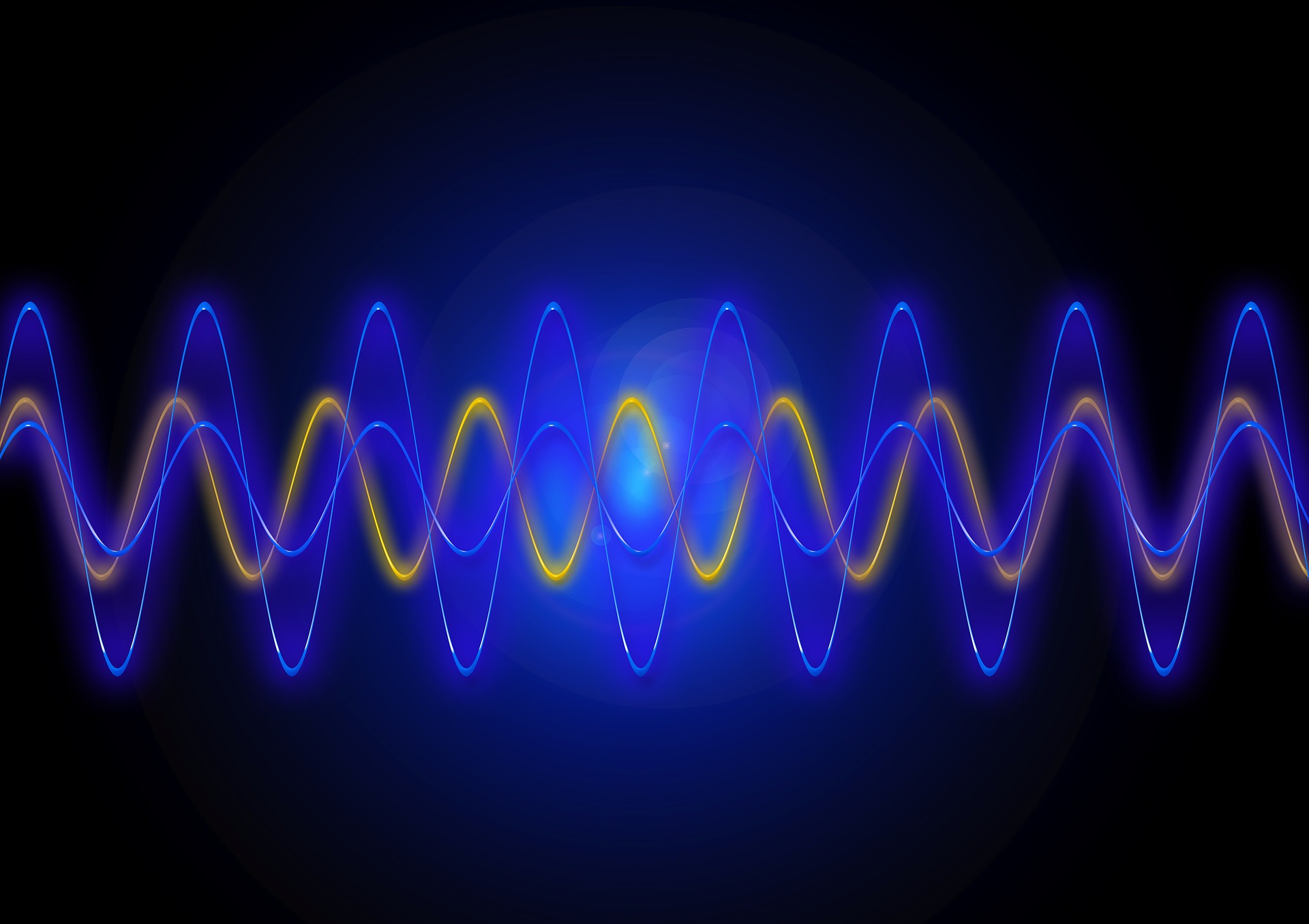
- DOI:
- 10.60864/bf04-9710
- Citation Author(s):
- Submitted by:
- Anonymous User
- Last updated:
- 17 February 2025 - 7:33am
- Document Type:
- Supplementary Materials
- Categories:
- Keywords:
- Log in to post comments
The emergence of text-to-image diffusion models marked a revolutionary breakthrough in generative AI. However, training a text-to-image model to consistently reproduce the same subject remains a challenging task. Existing methods often require costly setups, lengthy fine-tuning processes and struggle to generate diverse, text-aligned images. Moreover, the increasing size of diffusion models over the years highlights a scalability challenge for previous fine-tuning methods, as tuning on these large models is even more costly. To address these limitations, we present Stencil. Stencil leverages a large diffusion model to contextually guide a smaller fine-tuned model during generation. This allows us to combine the superior generalization capabilities of large models with the efficient fine-tuning of small models. Stencil excels at generating high-fidelity, novel renditions of the subject and can do so in just 30 seconds, nearly x20 faster than DreamBooth, delivering state-of-the-art performance and setting a new benchmark in subject-driven generation.