Documents
Presentation Slides
Super-Resolution in Compressive Coded Imaging Systems via l2 − l1 − l2 Minimization Under a Deep Learning Approach
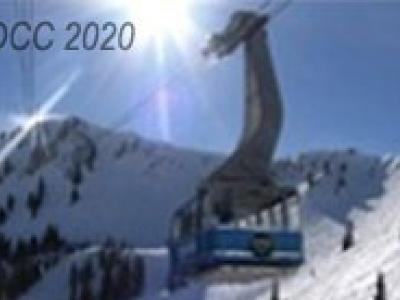
- Citation Author(s):
- Submitted by:
- Hans Garcia Arenas
- Last updated:
- 31 March 2020 - 4:47am
- Document Type:
- Presentation Slides
- Document Year:
- 2020
- Event:
- Presenters:
- Hans Garcia
- Paper Code:
- 184
- Categories:
- Log in to post comments
In most imaging applications the spatial resolution is a concern of the systems, but increasing the resolution of the sensor increases substantially the implementation cost. One option with lower cost is the use of spatial light modulators, which allows improving the reconstructed image resolution by including a high-resolution codification. In this paper, we propose a reconstruction methodology that exploits the intrinsic information contained in low-resolution measurements generated by the use of high-resolution spatial light modulators and high-resolution approximations obtained via a CNN. Specifically, based on a high-resolution CNN approximation, an l2 fidelity regularization term is introduced into a traditional l2 −l1 optimization problem. Finally, the simulations of the proposed l2 −l1 −l2 reconstruction approach show a quality improvement in up to 3.7dB in averaged PSNR against the use of the traditional l2 − l1 approach.