Documents
Description of Database/Benchmark
Supplementary for ICIP SF-VQA
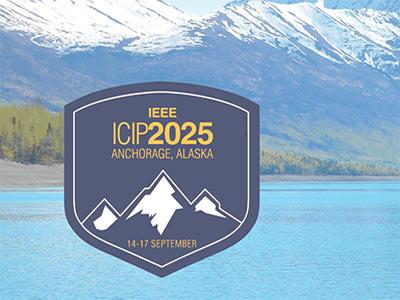
- DOI:
- 10.60864/49tx-n567
- Citation Author(s):
- Submitted by:
- Namuk Kim
- Last updated:
- 27 May 2025 - 7:37am
- Document Type:
- Description of Database/Benchmark
- Document Year:
- 2025
- Event:
- Presenters:
- Namuk Kim
- Paper Code:
- 1217
- Categories:
- Log in to post comments
Recent advances in deep learning have greatly improved No-Reference Video Quality Assessment (NR-VQA), with fragment-based approaches significantly reducing computational complexity through random patch sampling. However, challenges remain due to the reliance on random sampling and the uniform weighting of patches, which limit global observation effectiveness. To address these issues, we propose Saliency Fragments No-Reference Video Quality Assessment (SF-VQA), a novel method prioritizing salient regions. SF-VQA employs Saliency Grid Mini Sampling (SGMS) to select patches near areas of interest and utilizes the Saliency Grid Score (SGS) to compute a weighted quality score based on grid relevance. Experimental results demonstrate that SF-VQA achieves superior performance in Spearman’s Rank Correlation Coefficient (SRCC) and Pearson’s Linear Correlation Coefficient (PLCC) with minimal parameter increase, outperforming existing NR-VQA methods. This demonstrates SF-VQA’s efficiency and effectiveness in advancing NR-VQA.
Dummys.zip
