Documents
Description of Database/Benchmark
Supplementary Material: A Novel Context-adaptive Fusion of Shadow and Highlight Regions for Efficient Sonar Image Classification
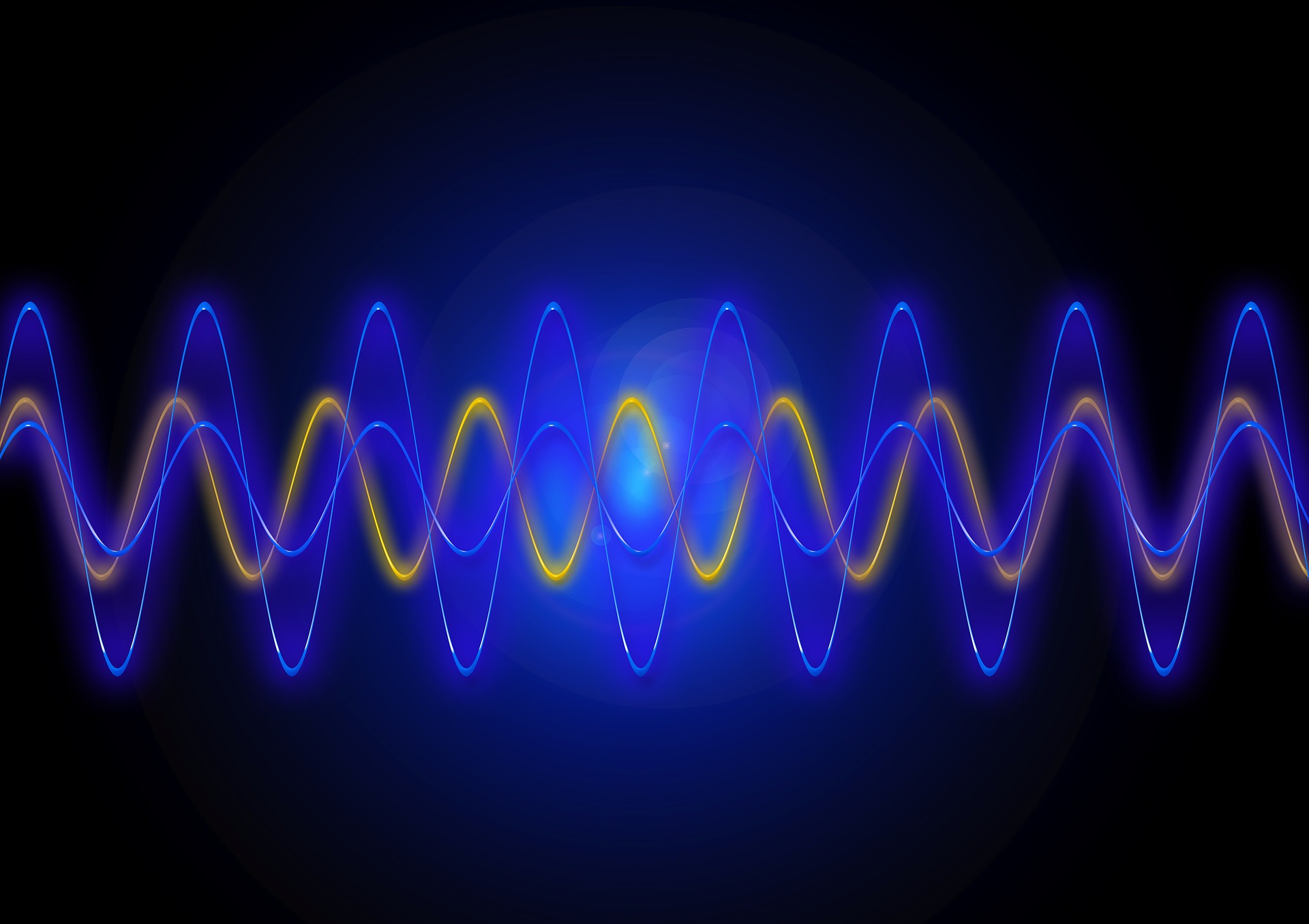
- DOI:
- 10.60864/yyh3-as08
- Citation Author(s):
- Submitted by:
- Kamal Basha S
- Last updated:
- 5 February 2025 - 5:19am
- Document Type:
- Description of Database/Benchmark
- Document Year:
- 2025
- Paper Code:
- 1801
- Categories:
- Log in to post comments
Sonar imaging is fundamental to underwater exploration, with critical applications in defense, navigation, and marine research. Shadow regions, in particular, provide essential cues for object detection and classification, yet existing studies primarily focus on highlight-based analysis, leaving shadow-based classification underexplored. To bridge this gap, we propose a Context-adaptive sonar image classification framework that leverages advanced image processing techniques to extract and integrate discriminative shadow and highlight features. Our framework introduces a novel shadow-specific classifier and adaptive shadow segmentation, enabling effective classification based on the dominant region. This approach ensures optimal feature representation, improving robustness against noise and occlusions. In addition, we introduce a Region-aware denoising model that enhances sonar image quality by preserving critical structural details while suppressing noise. This model incorporates an explainability-driven optimization strategy, ensuring that denoising is guided by feature importance, thereby improving interpretability and classification reliability. Furthermore, we present S3Simulator+, an extended dataset incorporating naval mine scenarios with physics-informed noise specifically tailored for the underwater sonar domain, fostering the development of robust AI models. By combining novel classification strategies with an enhanced dataset, our work addresses key challenges in sonar image analysis, contributing to the advancement of autonomous underwater perception.