Documents
Supplementary document containing experiment details
supplementary_1502_icip_2025
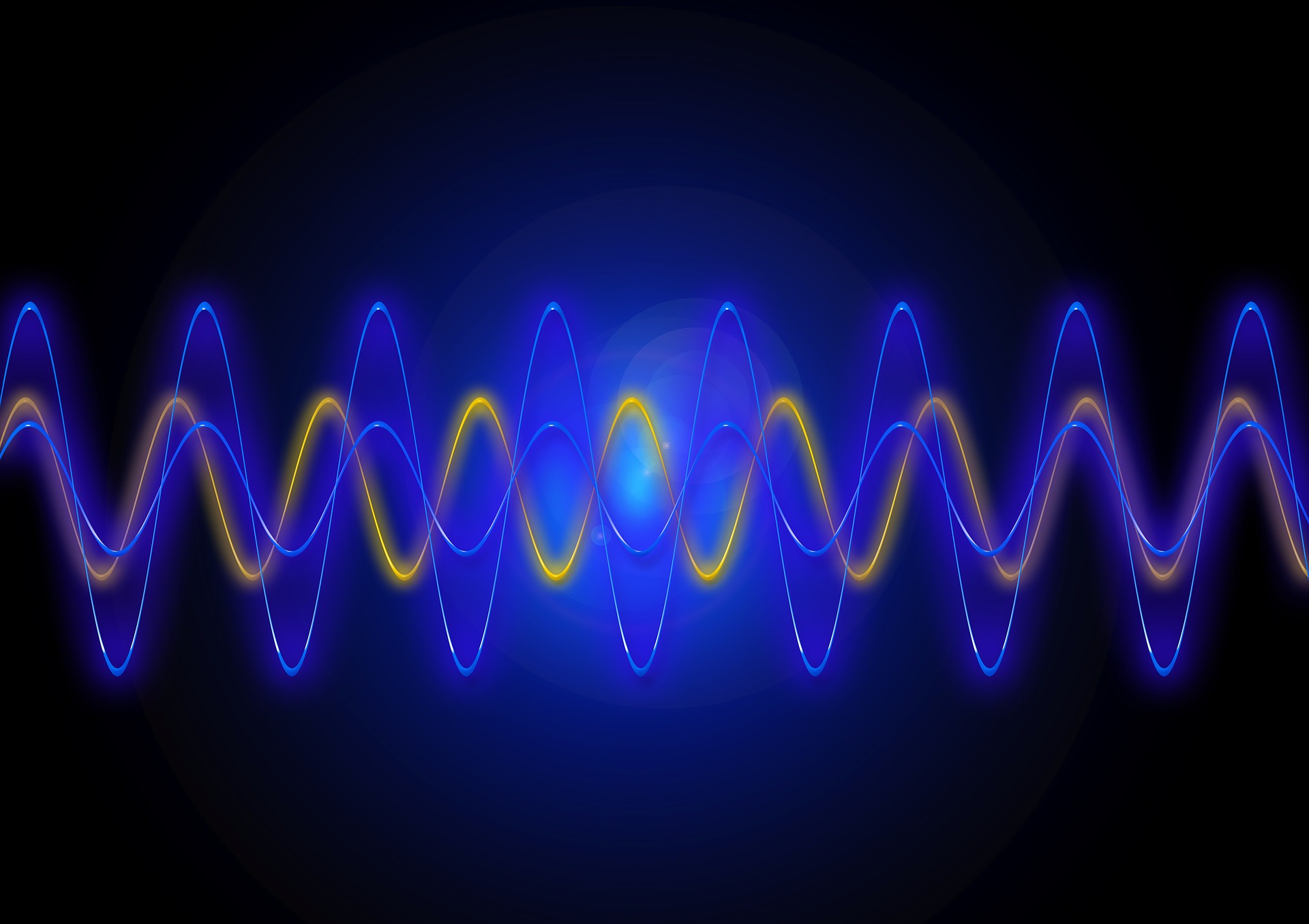
- Citation Author(s):
- Submitted by:
- Neha Bhargava
- Last updated:
- 21 January 2025 - 2:22am
- Document Type:
- Supplementary document containing experiment details
- Document Year:
- 2025
- Paper Code:
- 1502
- Categories:
- Log in to post comments
Machine Unlearning addresses the challenge of efficiently removing the influence of specific data from ML models, in response to data privacy laws and user requests for data deletion. Beyond simply excluding data from the training set, unlearning requires erasing its effects on the model parameters. We present a novel unlearning framework Unlogit, that uses sensitivity-weighted adjustments in model logits to forget selected data. Our multistep method involves: (1) identifying the logits that are most sensitive to the data to be forgotten, (2) applying sensitivity-based adjustments, and (3) optimizing unlearning with a dual loss strategy, minimizing KL divergence, and maximizing modified cross-entropy loss to target forgotten data. Our core approach operates without the need of retain-data, making it broadly applicable and efficient in various real-world scenarios. Our results demonstrate that the proposed approach significantly outperforms existing methods, offering a reliable and scalable solution for machine unlearning tasks.