Documents
Poster
Supplementing Missing Visions via Dialog for Scene Graph Generations
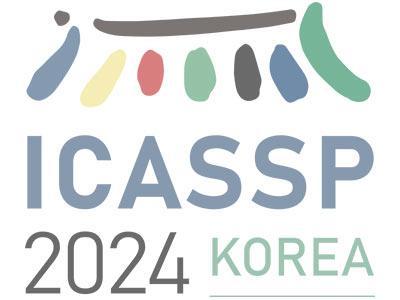
- DOI:
- 10.60864/4fhq-ek08
- Citation Author(s):
- Submitted by:
- Zhenghao Zhao
- Last updated:
- 6 June 2024 - 10:33am
- Document Type:
- Poster
- Categories:
- Log in to post comments
Most AI systems rely on the premise that the input visual data are sufficient to achieve competitive performance in various tasks. However, the classic task setup rarely considers the challenging, yet common practical situations where the complete visual data may be inaccessible due to various reasons (e.g., restricted view range and occlusions). To this end, we investigate a task setting with incomplete visual input data. Specifically, we exploit the Scene Graph Generation (SGG) task with various levels of visual data missingness as input. While insufficient visual input naturally leads to performance drop, we propose to supplement the missing visions via natural language dialog interactions to better accomplish the task objective. We design a model-agnostic Supplementary Interactive Dialog (SI-Dial) framework that can be jointly learned with most existing models, endowing the current AI systems with the ability of question-answer interactions in natural language. We demonstrate the feasibility of such task setting with missing visual input and the effectiveness of our proposed dialog module as the supplementary information source through extensive experiments, by achieving promising performance improvement over multiple baselines.