Documents
Presentation Slides
Presentation Slides
On theoretical optimization of the sensing matrix for sparse-dictionary signal recovery
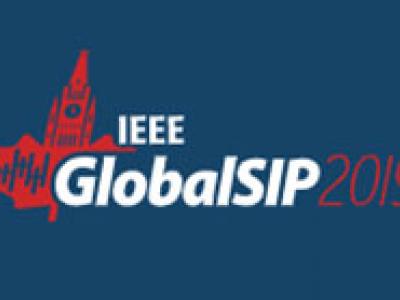
- Citation Author(s):
- Submitted by:
- Zhu Jianchen
- Last updated:
- 4 November 2019 - 10:52pm
- Document Type:
- Presentation Slides
- Event:
- Presenters:
- Zhu Jianchen
- Categories:
- Log in to post comments
Compressive Sensing (CS) is a new paradigm for the efficient acquisition of signals that have sparse representation in a certain domain. Traditionally, CS has provided numerous methods for signal recovery over an orthonormal basis. However, modern applications have sparked the emergence of related methods for signals not sparse in an orthonormal basis but in some arbitrary, perhaps highly overcomplete, dictionary, particularly due to their potential to generate different kinds of sparse representation of signals. Here, we first propose the Signal space Subspace Pursuit (SSSP) algorithm , and then we derive a low bound on the number of measurements required. The algorithm has low computational complexity and provides high recovery accuracy.