Documents
Presentation Slides
Time Difference of Arrival Estimation from Frequency-sliding Generalized Cross-Correlations Using Convolutional Neural Networks
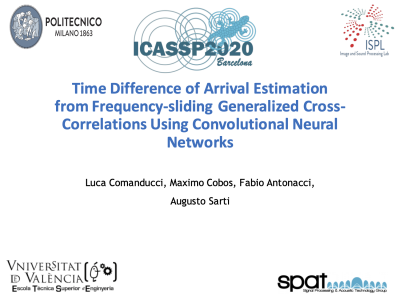
- Citation Author(s):
- Submitted by:
- Luca Comanducci
- Last updated:
- 20 May 2020 - 3:02pm
- Document Type:
- Presentation Slides
- Document Year:
- 2020
- Event:
- Presenters:
- Luca Comanducci
- Paper Code:
- SAM-P6.9
- Categories:
- Keywords:
- Log in to post comments
The interest in deep learning methods for solving traditional signal processing tasks has been steadily growing in the last years.
Time delay estimation (TDE) in adverse scenarios is a challenging problem, where classical approaches based on generalized cross correlations (GCCs) have been widely used for decades. Recently,the frequency-sliding GCC (FS-GCC) was proposed as a novel technique for TDE based on a sub-band analysis of the cross-power spectrum phase, providing a structured two-dimensional representation of the time delay information contained across different frequency bands. Inspired by deep-learning-based image denoising solutions, we propose in this paper the use of convolutional neural networks (CNNs) to learn the time-delay patterns contained in FS-GCCs extracted in adverse acoustic conditions. Our experiments confirm that the proposed approach provides excellent TDE performance whilebeing able to generalize to different room and sensor setups.