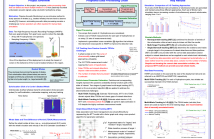
- Read more about PASSIVE ACOUSTIC TRACKING OF WHALES IN 3-D
- Log in to post comments
Passive acoustic monitoring (PAM) is a nonintrusive approach to studying behaviors of vocalizing marine organisms underwater that otherwise would remain unexplored. In this paper, we propose a data processing chain that can detect and track multiple whales in 3-D from passively recorded underwater acoustic signals. In particular, time-difference-of-arrival (TDOA) measurements of echolocation clicks are extracted from a volumetric hydrophone array's acoustic data by using a noise-whitening cross-correlation.
- Categories:
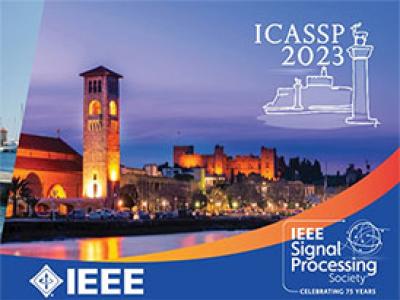
In-car child presence detection (CPD) has gained worldwide attention due to increased child deaths reported yearly when they are left unattended in a car. Existing solutions usually require dedicated sensors and are being surpassed by WiFi-based CPD because the latter can provide broader coverage and can reuse the in-car WiFi devices. However, the existing WiFi-based CPD solutions are not robust and may suffer from miss detection due to the very weak breathing of a young child and high false alarms under unfavorable environmental conditions.
- Categories:
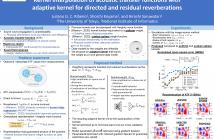
- Read more about Kernel interpolation of acoustic transfer functions with adaptive kernel for directed and residual reverberations
- Log in to post comments
An interpolation method for region-to-region acoustic transfer functions (ATFs) based on kernel ridge regression with an adaptive kernel is proposed. Most current ATF interpolation methods do not incorporate the acoustic properties for which measurements are performed. Our proposed method is based on a separate adaptation of directional weighting functions to directed and residual reverberations, which are used for adapting kernel functions. Thus, the proposed method can not only impose constraints on fundamental acoustic properties, but can also adapt to the acoustic environment.
- Categories:
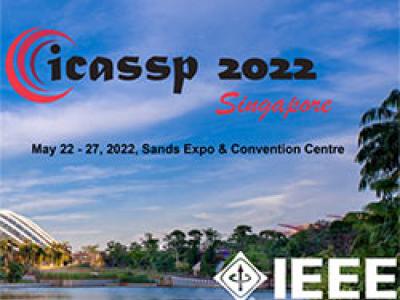
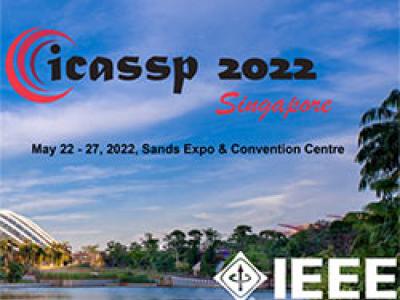
- Read more about JOINT SOURCE LOCALIZATION AND ASSOCIATION THROUGH OVERCOMPLETE REPRESENTATION UNDER MULTIPATH PROPAGATION ENVIRONMENT
- Log in to post comments
- Categories:
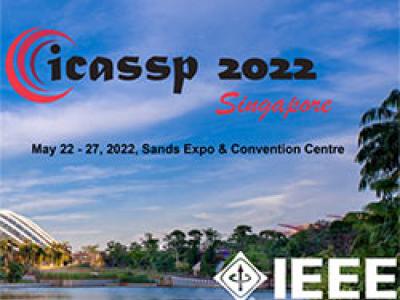
- Read more about Direct Localization: An Ising Model Approach
- Log in to post comments
Accurate indoor localization is a challenging problem in a multipath environment. In order to tackle this problem, several methods have been proposed. Direct localization is one of these methods that makes use of a two-dimensional search in a planar geometry. In this paper, we use a compressed sensing framework in the direct localization technique to estimate the location of a user in an indoor multipath environment. We form a penalized `0-norm structure for this problem, and then convert this structure to an Ising energy problem.
- Categories:
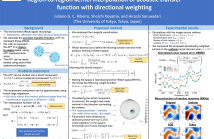
- Read more about Region-to-region kernel interpolation of acoustic transfer function with directional weighting
- Log in to post comments
A method of interpolating the acoustic transfer function (ATF) between regions that takes into account both the physical properties of the ATF and the directionality of region configurations is proposed. Most spatial ATF interpolation methods are limited to estimation in the region of receivers. A kernel method for region-to-region ATF interpolation makes it possible to estimate the ATFs for both source and receiver regions from a discrete set of ATF measurements.
- Categories:
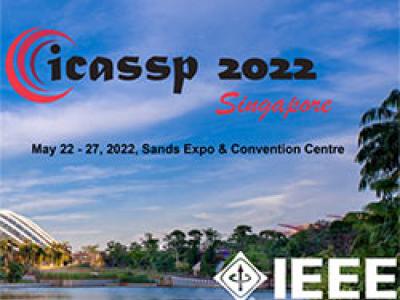
- Read more about Robust TDOA Source Localization Based on Lagrange Programming Neural Network
- Log in to post comments
This is the poster for the SPL paper: SAM-7.5: Robust TDOA Source Localization Based on Lagrange Programming Neural Network to be presented in the upcoming ICASSP 2022 conference in Singapore.
More details can be found at 10.1109/LSP.2021.3082035.
Thank you for your time.
- Categories:
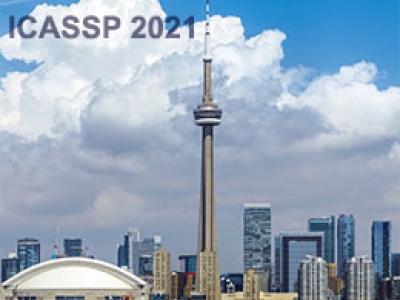
- Read more about FULLY-NEURAL APPROACH TO VEHICLE WEIGHING AND STRAIN PREDICTION ON BRIDGES USING WIRELESS ACCELEROMETERS
- Log in to post comments
Bridge weigh-in-motion (BWIM) is a technique of estimating vehicle loads on bridges and can be used to assess a bridge's structural fatigue and therefore its life.
BWIM can be realized by analyzing the bridge deflection in terms of its response to moving axle loads.
To obtain accurate load estimates, current BWIM systems require strain sensors, whose (re-) installation costs have limited their application.
icassp21s.pdf

- Categories:
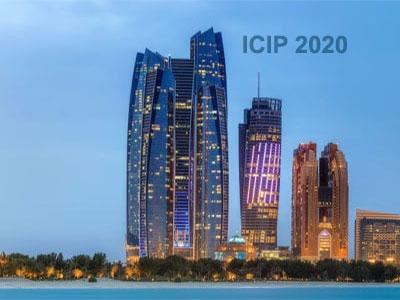
In this paper, a new robust principal component analysis (RPCA) method is proposed which enables us to exploit the main components of a given corrupted data with non-Gaussian outliers. The proposed method is based on the alpha-divergence which is a parametric measure from information geometry. The proposed method which is adjustable by the hyperparameter alpha, reduces to the classical PCA under certain parameters.
- Categories: